Published on
Data-Driven Decision-Making: Leveraging Predictive Analytics More Widely
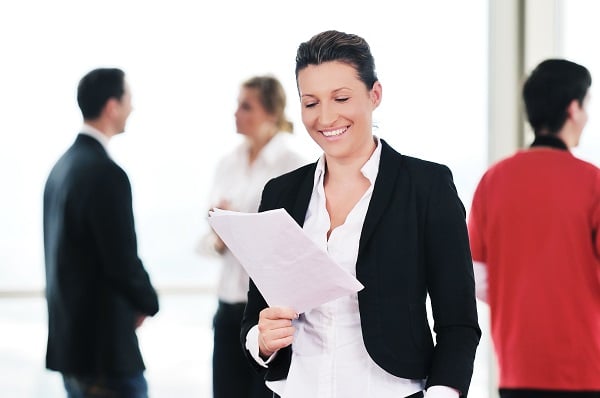
The hottest topics on higher ed campuses today are unequivocally student retention and student success. As college and university academics and administrators, we cannot tackle these challenges without getting deeper, much deeper, into the realm of Big Data, predictive analytics and data science. Our critical path to success in improving the student experience is to develop a laser focus on data-based decision making. We need to study the work of Thomas Davenport, of Michael Rappa, of George Siemens, and others. Colleges and universities are data rich and information poor. Few have any clue about the treasure trove of information in their existing systems; all they need do is transform the useful and toss the extraneous.
Data scientists can do just that. The real irony is that many of our institutions have that skill set right there within their own faculty. It is likely that these professors have never been asked to help. I recently asked a close colleague who teaches many courses in statistics in our College of Business if he considered himself a data scientist. Cold question. No lead-in. Without hesitation he replied, Yes. Follow-up. Is he on any of our myriad student success- or student retention-focused committees? No. Takeaway: Should he be? Absolutely.
In our own data, we can learn who has been successful and who has not. We can understand the students who enrolled, dropped out, transferred or graduated, and oftentimes why. We can learn where to concentrate our efforts, our passion and our resources. I contend that there is no way to accomplish that, to get to that point, without becoming heavily invested and highly skilled at predictive analytics.
What’s particularly fascinating is that we can see the issue of the use and expansion of predictive analytics in EDUCAUSE’s 2017 Top 10 IT Issues list. From information security to supporting student success to driving data-informed decision making (and, to that, improved data management) to the need for strategic institutional leadership and the demand for Next-Gen Enterprise IT, expanding the use of predictive analytics to support decision making and comprehension at all levels of the institution permeates this entire list.
How Predictive Analytics are Being Used Right Now
Institutions today are all over the map, as they say, when it comes to their leveraging of predictive analytics. Some are way out front, having engaged companies like Civitas Learning, and others are woefully behind, still trying to determine how to get started, oftentimes hampered by resource constraints. That said, at my institution, we have started down the right road with the best of intentions, are playing a bit of catch-up, and trying mightily to get where we should be. We need to go to the next level with this—and fast!
Technically, institutions using predictive analytics are looking at student characteristics and other KPIs, coupled with things like attendance and LMS usage. The LMS is often the key, where we can learn a great deal about our students. However, in order to mine that field rich with student data, the professors have to be using the LMS extensively and (almost) aggressively.
At some colleges and universities that is still the real challenge: increasing LMS adoption. Few academic leaders want to dictate this, but using the LMS provides useful data. It’s the linchpin for the successful implementation of predictive analytics in the learning space.
Leveraging Predictive Analytics Outside the Classroom
There are a few areas outside the direct teaching and learning functions of the university that could really benefit from leveraging predictive analytics. Enrollment Management and Academic Advising are the two administrative groups that come to mind first, as they would clearly benefit from having access to this kind of information. They would be able to then, in turn, support the students more and more effectively over time.
The library would gain, too, through valuable insight into student needs and, hence, be able to ramp up the part they play in student success and student persistence.
Ultimately, the acronym I have recently found most eye-opening in this context is RPG: Retention, Progress and Graduation. We should not remain focused only on the first two elements—we should have our eye on their prize: graduation! That is our end game, not merely continuing our tuition-based revenue stream. Every application of predictive analytics should be honed in on driving student success and helping them meet their goals.
Understanding the Limits
We all must keep in mind, however, that there are some limits to the use of data. One significant limit we must be aware of is the potential for profiling. We cannot allow predictive analytics to channel us to pursue only those students who will most likely be able to graduate. We also need to pursue those students who need to graduate, who our society and nation need to see graduate, and, who our world desperately needs to have graduate. Predictive analytics can be incredibly valuable in supporting students across the institution. We cannot use them to limit access. Instead, we need to use them to ensure expanded access does not negatively impact completion.
Roadblocks and Barriers
There are a few roadblocks leaders can expect to encounter when exploring predictive analytics options on campus. The first is the mindset that only consulting firms can aid us in this quest. We need to stop looking for the silver bullet, that one engagement or that single external partnership that will ensure our win in the student persistence and the student success realms. It’s important we recognize this is an ongoing process and requires thoughtful and strategic development.
That actually segues nicely into the second roadblock, which is the trepidation of starting out by leveraging internal resources—especially data scientists, who are already within our own faculty ranks—for what could be considered “experimental” work. Colleges and universities today are data rich and information poor. What’s more, we are resource constrained. We can meet and overcome both of those challenges by simply leveraging resources that we already have when it comes to predictive analytics. We just have to trust the process.
A Vision for the Future
Looking into my crystal ball, I believe the use of predictive analytics (PA) will become very common in ten years’ time—mainstream, even—at every level of every institution. From the president and provost to the student and TA, individuals across the institution will be able to fire up their personal PA Dashboard (with preset permission levels of course) to see relevant, contextual and near real-time numbers that will support their understanding of success. For students and student support staff, this could be depictions of how they are doing in their classes and how they’re expected to do. It could also show data on payment schedules and degree pathways. For senior leaders, this could show statistics on student and financial performance, and many other elements related to the student lifecycle in aggregate and anonymized form.
Simple, graphic dashboards will be key to the high-level understanding of this data, but the PA Dashboard system will also enable further query and investigation for those so inclined, through intuitive pass-throughs and drill-downs.
Everyone will have their customized look-and-feel that illustrates the information that they need—garnered from all of that back-end data that institutions have all been sitting on, and not truly leveraging, for so very long.
Students and instructors will be able to see, with one click, how they are doing in their specific section. Department heads will be able to see how folks are faring in their courses. Deans will be able to see how their college or school is doing in its programs—with drill-down capability to the section level. The Provost will be able to determine how the university or college is doing, with drill-down views through individual academic units to much more granular elements.
PA will become so commonplace that everyone will be making data-based decisions—on a routine basis.
It’s critical to note, though, that five to ten years is far too long a timeframe for such a system to exist, and for data-driven decision making to be commonplace at all levels. Frankly, no higher ed institution will take 10 years to get to this place, because they will already have ceased to exist by then.
Author Perspective: Administrator