Published on
Predictive Analytics and the Higher Ed Overhaul
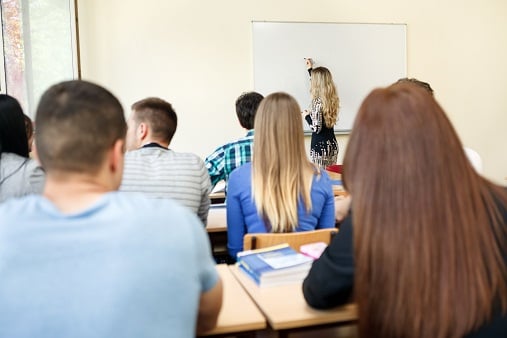
An early adopter of predictive analytics was Purdue University. Signals, Purdue’s predictive software, was developed in 2005 to detect students struggling in their courses.[2] The software provided early detection and a warning signal generated and sent to both student and faculty. Interventions with suggested resources (tutoring, faculty office hours, etc.) followed the warning signal in an effort to address the identified challenges.
Rio Salado College developed a similar predictive system, RioPACE, that uses student historical data from Rio’s proprietary learning management system. Student historical data is then compared to current student behaviors focused on course log-ins, lesson engagement and progress on assignments. These behaviors are then categorized and provided to students and faculty, letting students know if their behaviors are on target for success or if they need improvements in one of these three areas. Interventions and communication are generated from the faculty to pinpoint areas where students are struggling, either on online behaviors or more specific content assignments. RioPACE also gives the instructor a snapshot of how students are behaving in the online course.
Predictive analytics, regardless of the algorithm behind the model, can increase instructor immediacy in an online classroom. Students enrolled in online programs can often feel isolated and struggle with how to manage the work load in an online environment. Analytics can help students create online behaviors that lead to success. Students are able to see their progress in an online course either compared to current or historical students. Were students logging in more often, were they engaged in the online lessons more frequently or for a longer period of time? It creates a learning tool to improve online learning behaviors.
Instructors have a snapshot of how engaged their students are in the course. It provides a summary of their activity, use of the content and connection to their grades on assignments. Instructors can intervene with early detection and help students get back on track. Interventions may include a phone call to help increase student motivation and decrease isolation or content-specific resources related to the lesson objectives.
The possibilities are endless; the focus is on student retention and success by improving students’ online learning behaviors.
– – – –
References
[1] Marc Parry, “Colleges Mine Data to Tailor Students’ Experience,” The Chronicle of Higher Education, December 11, 2011. Accessed at http://chronicle.com/article/A-Moneyball-Approach-to/130062/
[2] David Raths, “Learning analytics efforts apply business intelligence to student retention,” KM World, April 29, 2012, Vol. 21 (5). Accessed at http://www.kmworld.com/Articles/Editorial/Features/Focus-on-KM-in-higher-education–Learning-analytics-efforts-apply-business-intelligence-to-student-retention.-82034.aspx
Author Perspective: Administrator
Author Perspective: Community College