Published on
What Every College Administrator Should Know about Predictive Analytics
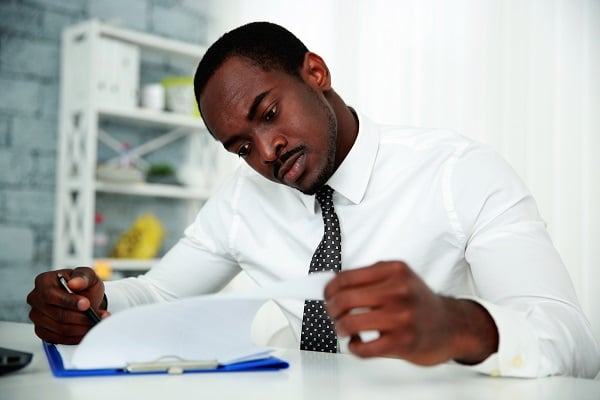
Predictive analytics are making their way onto college campuses. 40 percent of colleges report using this data on campus. Schools that are using predictive analytics are identifying and preventing problems before they happen, rather than after the fact. The most common uses of predictive data are to help institutions meet enrollment or student success goals.
Predictive analytics can help colleges determine which students they should aggressively recruit. Or, how to personalize each student’s academic experience once they enroll to help make sure they stay in college and finish their program. Take Georgia State University’s use of predictive analytics. A public research university in Atlanta, the university uses predictive analytics to pinpoint students who are at risk of steering off track in their programs. In one year alone, the university was able to convene over 51,000 in-person meetings between students and their advisors. Over 51,000! By increasing their advising staff and centralizing their advising system, Georgia State is the only university of it’s type to have pulled off something else pretty amazing: eliminating the achievement gap for low-income, first-generation and students of color.
Despite the success at Georgia State, it still may be difficult to overlook the ethical concerns with using predictive data. For example, how to ensure colleges don’t discriminate against students of color and low-income students. How they will keep student data private and secure. How institutions can build and use predictive tools as transparently as possible.
Here are some myth-busting facts to help crack the code on the benefits and dangers to using predictive analytics in higher education.
Myth 1: Predictive data in admissions processes results in colleges weeding out harder-to-reach students.
Facts: Admissions teams use predictive data to determine which students have a good chance of enrolling at their institution and are, therefore, worth aggressively recruiting. Students with high predictive scores tend to be students who a university has enrolled successfully in the past. This can mirror and exacerbate inequities we now have in society: Being affluent and white puts you at the top of any list worth being on. But it doesn’t have to be that way.
Georgia Southern University, a public research university, uses predictive analytics—as you might expect—to make strategic use of limited recruiting resources and time to communicate to students who are likely to enroll. Georgia Southern doesn’t focus on students with high predictive scores (meaning their chances of enrolling are promising). The university excludes—yes, excludes—these students from targeted outreach. But they don’t stop there. The university re-directs its attention and resources to students with lower predictive scores, but whom they believe may successfully enroll at Georgia Southern if given the proper chance.
Myth 2: Because data is neutral, it cannot lead to discriminating against certain students.
Facts: When we aim for neutral decisions, what we’re really after are objective ones. No technology is devoid of values or biases, nor are the people who use them. Therefore, technological solutions cannot be neutral. No matter how hard the try.
Unfortunately, the same goes for data.
Data is generated by people, institutions and processes. None of these things are or can be “neutral.” Predictive analytics rely on an institution’s historical data to make their predictions. Historical data carries with it all the things that went right and wrong at an institution.
Consider for example an institution that hasn’t done a particularly good job at graduating low-income or students of color who pursue STEM majors. Predictive systems built with this institutional knowledge will take this into account—perhaps reasonably so—when considering which students in STEM programs should be flagged as at-risk.
However, colleges run the risk of allowing predictive analytics to single out, marginalize or profile underrepresented students. This is especially so when comparing these students to the overall student body. This could result in underrepresented students who may or may not need support getting singled out, potentially in a negative way. And, students who don’t “fit the description” get overlooked for support they very well may need. It’s a lose-lose situation either way. Predictive data should propel colleges to become more nuanced, not less, about what risk does and doesn’t look like at their institution.
Myth 3: As long as a college complies with FERPA, predictive analytics don’t raise any new privacy concerns.
Facts: FERPA is the privacy law governing how students’ educational records are shared, reviewed, and corrected for errors. Much of the data colleges use or create with predictive analytics can be included in a student’s educational record. For example, sharing in real time data or notes from appointments can help staff change the trajectory of struggling students.
The problem with just complying with FERPA when using predictive systems or being innovative on a campus is that FERPA alone may not protect your institution or students. It just simply hasn’t kept up with the times.
Our technologies and even processes—like sharing data with those inside and outside an institution (E.g. vendors)—have become more commonplace and more complex. As a result, colleges are left with more questions than answers about how to protect student privacy in this new environment and remain in compliance with FERPA. This only means colleges will need to become more vigilant about ensuring students’ data privacy is protected. Good places to start could be getting students’ consent to use their data for predictive analytics and ensuring that students’ personally identifiable information is obscured when sharing data to be later analyzed by vendors or researchers.
Myths are powerful forces, especially when they contain some truth. However, we owe it to ourselves and our students to let facts guide our work and visions for the future.
Author Perspective: Analyst