Published on
10 Steps to Establishing a Data Driven Campus Culture
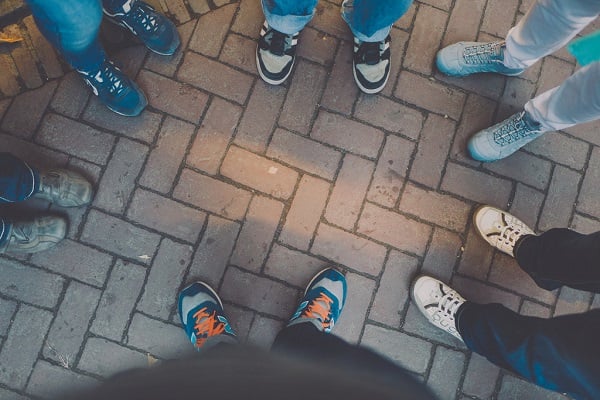
In many ways, higher education has been late to the game of data-informed decision making. But there is undeniably a recent groundswell of interest, particularly to inform program design and student success efforts. We all want students to succeed, and most of us in higher education have worked diligently toward that end for decades. We have hired consultants, implemented all the known best practices, measured outcomes, surveyed students, evaluated our efforts, and implemented feedback loops.
Having done all of this, many institutions have plateaued, fighting harder for further gains in retention and graduation rates. There are still students who don’t complete their degrees but who could with the right support at the right time. How do we reach those students? How do we determine those next steps? How can we target interventions where they are needed most, when they are needed most? The answer is in one of our most abundant resources: data.
We live in a data-rich world and the tools for analyzing, interpreting and formulating action from that data are increasingly accessible. Think about the ubiquity of data-informed decision making. Algorithms help us buy products based on recommendations for “people like us.” Our banks provide analytics of our spending and investment performance. We track our movements to improve fitness. We are accustomed to having data at our fingertips in order to make all manner of decisions.
Many campuses now have data warehouses and self-service analytics environments, and with that comes the expectation at all levels of management to use it. Those expectations have evolved beyond standard reporting; the call now is for strategic data to inform action. There is more demand for predictive analytics, scenario testing, optimization modeling and the like, the types of methods necessary to target our limited resources for maximum impact. More of us are using data to inform decisions because, simply put, we must.
But implementing systems for analytics is just the tip of the iceberg. Colleges and universities are big places, often decentralized, where success depends on activity at all levels. So any change truly takes a village, but simply peppering that village with data will not lead to better decisions or action. Once analytics and data insights have been developed, members of the campus community have to engage in a process of interpretation to collectively assign meaning to it. From there, planning and action can take place. Many institutions are now deploying analytics platforms that promise not only to create new insights, but also to deploy those insights to various actors, including institutional researchers, faculty, advisors, and student success personnel, each of whom can then engage in their own data-informed decision making. We learned that in order to facilitate distributed action, we had to present data in the best interest of all parties involved.
Everyone at the university can use a little intel to help them support students. For instance, faculty can benefit from analytics about their students’ engagement with course materials, alerts about under-performance on milestones, or other indicators of performance. If you were a faculty member, wouldn’t you appreciate analytics that could help you differentiate between a student who is working hard yet underperforming versus a student who is not performing and not investing enough effort? This is the kind of data that can make their lives easier and their pedagogy stronger.
The same is true for academic advisors or student success personnel who may be responsible for large case loads of students. Precious time with a student can be saved by having all relevant data about the student in one place, rather than hunting for it in multiple systems of record. Dashboards with key analytics related to the work of advisors (registration, financial holds, log-in info for learning management systems, low midterm grades, etc.), enable advisors to more easily engage in targeted outreach that is personalized, timely, and topic-specific. We must use data in a way that empowers our frontline student interactions.
There are, however, some misconceptions about using analytics in a data-informed culture. One concern is that data such as predictive analytics is deterministic, that data labels students as numbers and therefore limits their options or aspirations. But the identification of a student within a trend, historical pattern, correlation, or predictive factor does not equate to a sealed fate. In fact, by identifying a risk factor early, we create the time, space, and insight to influence a student’s outcome for the better. What historical trends and predictions show us is probability and by working with the data to identify that probability we have the chance to make a difference for that student.
Another misconception is that data can tell us everything. In fact, the things we learn in our analytic adventures are actually just signals that help us focus our attention on a particular student group, business practice, academic policy, or aspect of the curriculum where risk or opportunity exists. Any signal generated by predictive analytics requires interpretation and investigation. Good data systems can crunch a whole lot of information, systematize it, present it in a format that is easy to navigate, and signal areas for further exploration. Data can tell us where to look more deeply, but they do not diagnose the problem in most cases.
Creating a data-informed culture at every level of a campus is a real change management challenge. Here are a few basic suggestions to help ensure that the culture change endures:
1. Do not boil the ocean. Identify the problems you are trying to solve and prioritize them. For every insight you hope to deliver through data, there must be due process before it routinely informs decision making, so you want to stay focused.
2. Implement a schedule for data delivery that aligns with critical times for planning and action throughout the year. Identify inflection points: When can you take action to build momentum towards your outcome? Where might you lose ground? How can data help you minimize losses and maximize momentum?
3. Institutionalize expectations for data to be collectively reviewed with stakeholders. This can and should happen, albeit differently, at all levels including large summits, cross-functional tiger teams, and program-level all-hands meetings.
4. Keep a conversation going about how you will continue to learn new things. Are there additional sources of data that should be integrated into your analytics? Assess your data readiness and start working with campus partners to get there.
5. Resource your analytics efforts appropriately for the long term to ensure that your teams can maintain and improve them over time as changes occur in technology, systems, policies and business practices.
6. Deliver data in a way that is easily understandable and that supports workflows, helping people move smoothly from information into action.
7. Data requires data literacy, so plan for sustainability with learning opportunities. Create and support user communities to facilitate shared learning.
8. Stay close to those who use the data. Get their feedback, understand how the data is or is not working for them, keep them in the loop about the effectiveness of their efforts, show them that their feedback matters, and listen for the evolution of their needs.
9. Measure your efforts. There is no way to gain trust in data except to provide evidence of its accuracy. Review projections against actual outcomes and assess the effectiveness of programs and interventions.
10. Celebrate your successes! Recognize those individuals who have made positive change through data-informed decision making and position them to help and influence others.
Many of the suggestions above reiterate the fact that a successful data-informed culture is people-centric. We need to use our data more effectively in order to take the higher education enterprise to the next level, but systems and algorithms are just the beginning. Our data is about real people, the outcomes we seek are life changing, and the only way data works is through the web of understanding and action we collectively create as colleagues.
Author Perspective: Administrator