Published on
Driving Institutional Change with Data: Identifying Obstacles to Actionable Business Intelligence
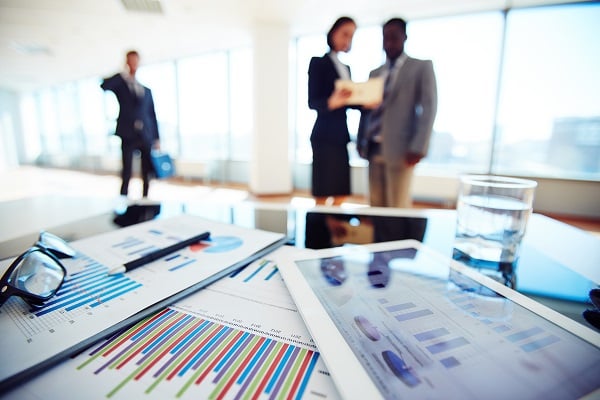
When building an in-house business intelligence division, creating a campus movement behind leveraging data to support decision making and institutional strategy is arguably the “easy” part. What’s left is actually turning information into actionable outcomes, and that can be immensely challenging. From understanding and responding to the requirements of a broad range of stakeholders to ensuring the cleanliness and consistency of data being analyzed to sharing the data in the most effective way possible, there’s a lot that goes into making actionable business intelligence a reality. In this interview, Hank Childers reflects on the path his team took to get their business intelligence work off the ground and shares some insights into the challenges and obstacles other leaders might face.
The EvoLLLution (Evo): What are some of the most significant mistakes higher education leaders tend to make when launching business intelligence projects?
Hank Childers (HC): One mistake higher education leaders tend to make when launching business intelligence projects is to become too wrapped up in the technical aspects. A business intelligence project is really a business project with a technical component. However, it is tempting to focus on the technical aspects, because they appear to be the primary barriers to success, and seem to represent the biggest apparent gap between where one is and where one wants to be. For example, there is a rich body of thought and practice around dimensional modeling, and a language that goes with it. If the organization is not already familiar with these concepts, then this may seem like the primary barrier to getting where one wants to go. In teaching business intelligence, I emphasize the importance of understanding these key concepts, but using language that truly communicates and focuses attention on the underlying business processes and goals.
Another mistake is to act as though we already understand the requirements well, and thus we can and should get it right the first time. For example, at Arizona we built a set of models and dashboards/reports around the needs of research administration, based on data captured in the research administration transaction system. It provided a set of information that had not previously been available. However, after two or three years, we realized that we needed to go back to the fundamentals and redesign the dimensional models, based on a better understanding of the requirements, both on the BI team’s part and the client’s part. It was a difficult decision, because it seemed like a step backward, but in fact, it was a big step forward. The second version is both dramatically simpler and substantially richer in the ways that matter, which we now understand better. While we talk about requirements as a fixed deliverable, it is arguably as much a process and an ongoing evolution. The world changes, and the way we look at that world changes.
Evo: What does it take to define the most important data for an institution to collect?
HC: Defining “the most important data” is difficult, because there are multiple constituencies. In earlier days, we believed that business intelligence (or decision support) was something for primary business decision makers only. However, now we see that decision-making spreads out both across and up and down the organization. Moreover, the distinction between reporting, dashboard, and analytics is better seen as a continuum. We average close to 30,000 queries a day in our BI environment, with nearly 2,500 distinct users per month. Supervisory and middle management layers initially drove the development of our BI tools, and this kept us very busy for a long time. The development of these layers also served to validate the data and establish confidence in it as a foundation.
Over time, we turned our attention more to the needs of senior administration. However, this in turn exposed the fact that the world of business intelligence and the world of institutional research needed to be aligned, or we could not be successful. I think this alignment (either organizational or operational or both) is a critical success factor.
When BI practitioners inquire about how to identify the most important data, I usually urge them to “wrap yourself around the questions that matter most to the institution’s senior leadership.” In practice, this means some kind of seat at the table, not so much as a decision maker, but in terms of awareness. It also means looking outside the institution to the issues that matter to other institutions, and the forces that are at play.
While this question asks what data is important to collect, it is probably more accurate to speak in terms of what data is important to curate. Most data collection happens as an adjunct to business processes that are already in place to serve transactional needs. We can also obtain some information from external sources, e.g., for benchmarking. However, in practice it is usually very difficult to identify a new data need, and then set out to collect it on a sustainable basis, unless we tie it to an already existing business process.
Evo: How challenging is the process of cleaning data to make it useful for analysis?
HC: The task of cleaning and preparing data can be daunting and seemingly never-ending. It involves not only managing the reality of data quality, but also the impression that people have of the quality of the data. Yet if we break this mountain down into smaller chunks, we find that we can make things better, and in doing so provide real value. Here are a few core issues related to the problems of cleaning data:
The number one problem is probably lack of agreed-on definitions and readily available documentation of the definitions. For example, when discussing student enrollment, is the quantity based on headcount or FTE? When discussing number of faculty, whom do we count as faculty? The answer to these questions is the famous consultant response: it depends! That is, it depends on context. Most of the data governance initiatives that I have seen have focused on data definitions, and associated priorities. This is not easy—there are literally thousands of potential data items—but it is amenable to steady improvement by focusing first on the elements that matter most.
The number-two problem then is likely to be differences in the currency of the data. The transaction system number will very often differ from the data warehouse number, because the former is real-time, while the latter is end-of-day. It is interesting to note that these two problems do not involve data being wrong, but being wrongly understood. (Or perhaps wrongly presented.)
The next two problems, though, take us into the data itself. The first of these has to do with consistency across different source systems. Do all of our systems use the same definition for department, for college, for account, for employee, for building and room, etc.? These are among the primary ways we have of organizing and grouping data. Having ERP systems in place helps a lot, but even in that case these systems are often governed differently and are subject to different forces. Thus, they tend to drift apart over time. It is tempting to think that these identifiers might be different between one system and another, but they can still be cross-referenced. However, it is usually not that simple. Department X as seen from the financial system may not be quite the same as department X as seen from the student system. Centralized management of these key identifiers is key. This is perhaps the other primary responsibility for data governance. When things drift apart, it is much more difficult to bring them back together.
The last problem is probably the one we think of first when we think about data quality—sometimes the data is just wrong. For example, the local student address is wrong for a given student. How does that happen? Actually, it happens all too readily. Most of the data with which we concern ourselves is data captured in one of our transaction systems such as Human Resources, Financial, Student, etc. This data is captured as part of a business event such as hiring, procurement, registration, etc. The data quality then is inherited from the business event that occasioned its capture, and the arbiter of quality is thus the business event itself. Unless that business process somehow utilizes the data it captures, or has a verification step, the quality will suffer, because the business process will proceed just fine even though the data is wrong. Another common occurrence is that the data may have been accurate when it was initially captured, but it changed later, and there was no business event transaction associated with that change.
It is interesting to note that serious data quality problems can exist for years without being realized, that is until we try to do something with that data. In fact, one of the very effective approaches to improving data quality is to expose that data to view. When people see that it is wrong it can rally forces to fix it. These efforts take us from the business intelligence world back into the world of the transaction systems. It is important that this path be open, even though it inevitably involves crossing one or more organizational lines. This, too, is where cross-organizational data governance can make a difference.
As a final thought, I think that data quality is probably best assessed as a question about fitness for its intended use. Precision matters more in some contexts (such as IPEDS reporting), while in other contexts a degree of approximation is acceptable (such as the number of on-campus parking spots needed to handle the incoming class).
Evo: Why is it important for an institution to have data analysts specifically focused on collecting and analyzing data, rather than leaving this work to programs and faculties?
HC: Increasingly the data elements that matter to us cut across organizational lines, and need to be viewed as an institutional asset. This is true of student data, employee data, faculty data, financial data, research data, etc. At the same time, we are looking more and more to integrate data across these realms. Consider, for example, the creation of a department profile that includes all of these types of data to support comparative analysis. It is not practical to do this with a decentralized approach, since the data must be consistent and integrated. A centralized or hybrid approach makes the most sense.
In addition, some of the skills needed to do this work are specialized, such as dimensional modeling, ETL, and data science. This is particularly true around the creation and maintenance of the central data warehouse (or equivalent) and the application of data science techniques. There is definitely a need for subject matter experts and other analysts to contribute meaningfully to the effort. Some institutions choose to keep all of this centralized. My view is that many people coming into the institutional workforce are already very savvy about data, and will effectively demand access so that they can do their own analyses. Thus, a hybrid approach makes sense to me.
Evo: Ultimately, what advice would you share with postsecondary leaders looking to launch a data analytics initiative?
HC: In much of our lives, our society treats data and information as a free good. Our expectation is that it is out there, and we can avail ourselves of it with little if any expense required, other than the time it takes to find it and get familiar with it. This is similar to how we see the library. For most of its users, it is a free good, but from an institutional perspective, it requires a significant and sustaining allocation of funds. Business intelligence and institutional research are in a similar position. We can look at them as an expense, or as an investment in data. In these changing and challenging times, I think investment is the better approach. We need to manage this investment appropriately, with the help of the central and extended teams.
Author Perspective: Administrator