Published on
Analytics and the New Academy
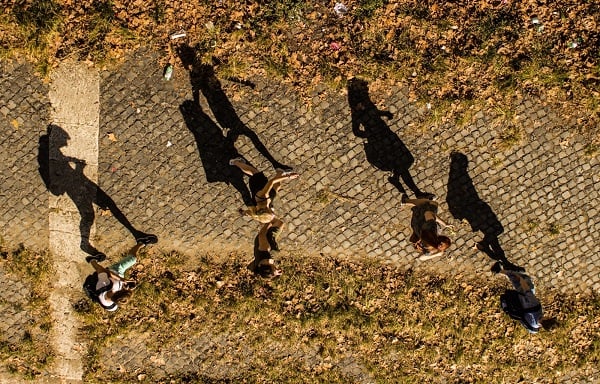
Analytics! In higher education, seeking meaning in patterns only observable from a distance is changing the way we see and know our students. Blurry clusters of dots—whether in student demographics, behaviors or circumstances—can provide a broader understanding. Those individual dots in our data now form observable trends through a lens of pattern matching. Just as Seurat and Signac allowed us to understand images differently, analytics work provides a new data pointillism (Carmean and Robinson, 2016), by displaying the dots in previously unseen ways, and enables institutions to expand what can be known about our learners.
This is, of course, only possible because we collect massive amounts of data. We always have, but we now sort, discover, process and predict based on that real-time data. This began with moving much of our university data into a common, user-friendly container visible through interactive Tableau reports.
The University of Washington recently went live with Civitas Learning to explore domains of prediction for student retention, performance and success. Pulling together data that has previously sat in closed silos – SIS demographics, Financial Aid, LMS performance and engagement, enrollment patterns, quarterly grades and more – cloud-based joins make the data points available in one connected interface. Analytics allows us to plot persistence based on data long hidden, serving as powerful predictors of student success.
The Challenge
We can now know, but what do we do? The challenge, we find, is the “obligation in the data” regarding having the tools, using them, and taking action based on what is found. The work of discovery demands a way of looking at work that is new to many who are now asked to take on the role of analytics worker.
This realization has been hard for our campus, The University of Washington Tacoma, to understand and acknowledge. Why? This is new knowledge, not of historical numbers, but of our current students.
To understand this work, let’s start with what analytics is not. Analytics is not:
- There are no key reports with totals to display.
- Data analysis. There are no counting, dividing, summing or pie charts to view.
- A tool that tells you why. After hard discovery work, you find out that a sector of students do better or worse than their peers, but the patterns don’t tell you why.
The Experiment
Over the course of a year, with new tools in place, UW Tacoma has dug in, tried, failed and adjusted our framework for analytics work. First, we looked to Institutional Research to lead the way, but early on recognized that professionals very good at counting, reporting and analyzing data are not suited to the work of searching for insights. #FAIL
Next, we formed a weekly work group of leaders across the campus who understood the value of analytics. We did not consider the research on the propensity for quick results work in people in leadership positions, and how frustrating data discovery work can be for this population (see Glazer, 2015). Like IR, this group soon became frustrated with the time it takes to search for insights and stopped attending. #FAIL
To help us regroup and move forward, we brought in a consultant, Randy Swing, who served as Executive Director of the Association for Institutional Research (AIR) from December 2007 to January 2016. Together with Randy, a team of people explored new ways to create a data culture, how to better understand collaborative data sharing, and to create new models for doing analytics.
Finally, we were ready to look at ways to change if we were to successfully form a “federated network” for institutional research work that leverages local, program-level curiosity and questions (Swing & Ross, 2016). Our UWT Program Data Analysts focus on their own students, interests and goals and work independently to contribute to greater insights. Within this model, Institutional Research is responsible for training, general population insights, and institutional reporting and research. Local programs explore their own data, share insights, and determine success for their students.
So what does analytics work look like? Frustrating and fascinating. Time consuming and game-changing. Work infused with the obligation of knowing and keeping populations discovered as newly vulnerable on course. Work that allows us to see, in real time:
- The tipping point for attrition is not pre-registering 20 days before quarter starts.
- Self-pay students are our most vulnerable population.
- Students who take one online course at our urban-serving campus have a 3-7 percent higher retention per quarter than peers that take all on-ground courses.
- 70+ percent of attrition are students in good academic standing.
A Federated Model
Local goals, community-built skills, and shared insights. UW Tacoma is finding that analytics changes the way we see and know our students, and unlike traditional reporting, calls for a data culture that recognizes:
- The aptitude of a worker that digs deep on discovery, is patient with pattern-matching and sees the value in new, visual knowledge stores.
- Need for a community-based data culture focused on sharing insights aimed at local results
- A federated, consensus-built data culture working with leadership to create new strategies and supports for our learners.
- Shared goals, new resources for discovered insights, and the obligation of knowing.
This has been the University of Washington Tacoma’s year-long experiment in analytics work. Connection between discovery, mapping, and the challenge of the aggregate. We’ll keep you posted on what comes next.
– – – –
Further Readings
Carmean and Robinson (2016): Predictive Analytics: Moving from Data to Decisions http://www.slideshare.net/carmean/analytics-from-data-to-decisions
Civitas Learning Space. https://www.civitaslearningspace.com/
Glazer, Jessica (2015). ADHD Can Be a CEO’s Secret Superpower. http://www.huffingtonpost.ca/jessica-glazer/adhd-ceo-career_b_8124154.html
Swing, Randy and Ross, Leah Ewing (2016). A New Vision for Institutional Research. https://www.airweb.org/Resources/ImprovingAndTransformingPostsecondaryEducation/Documents/A-New-Vision-for-Institutional-Research.pdf
Author Perspective: Administrator