Published on
Humpty Dumpty, AI and the Mastery of Meaning: Navigating Bias in a Digital Age
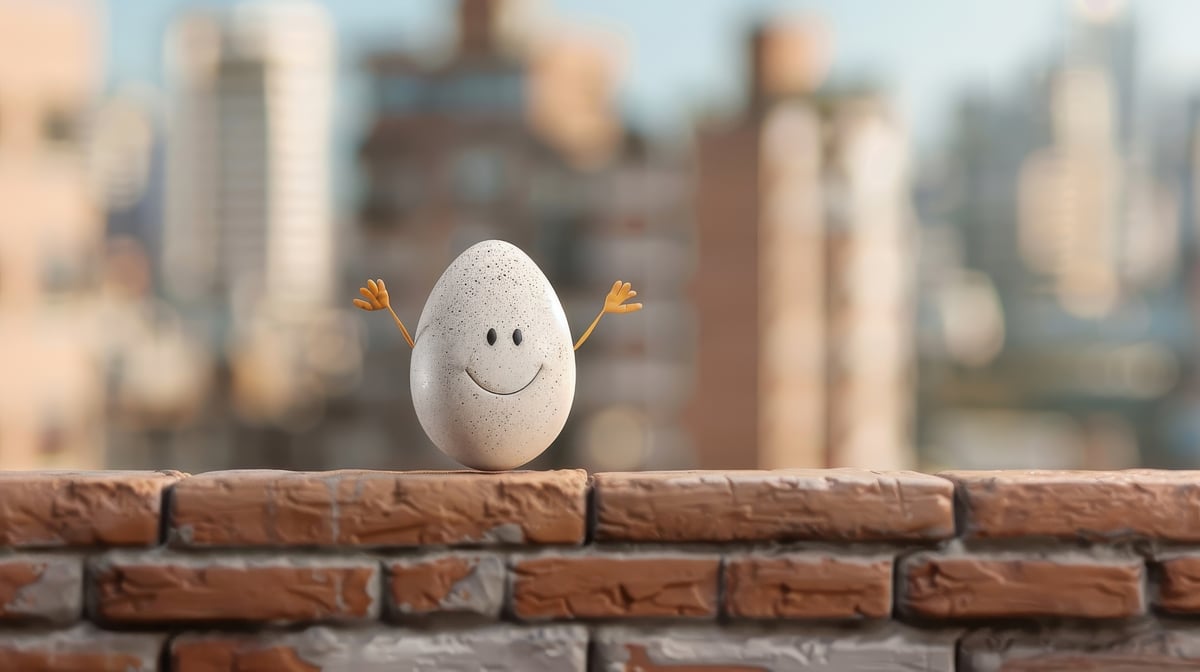
Humpty Dumpty famously declared to Alice, “When I use a word, it means just what I choose it to mean, neither more nor less.” When Alice protested that words surely could not hold infinite meanings, he calmly but pointedly replied, “The question is, which is to be master, that’s all.” Lewis Carroll’s whimsical dialogue encapsulates a profound philosophical tension: meaning, truth and reality are never completely neutral or objective. Even if an unbiased reality existed, our act of observing it would inevitably be shaded by our own filters, biases ingrained through experiences, education and cultural conditioning.
In the contemporary world, artificial intelligence (AI) powerfully mediates our relationship to knowledge, in some cases more deeply than human teachers, journalists or other traditional gatekeepers. AI’s new role underscores the unsettling resonance of Humpty Dumpty’s claim. AI’s unprecedented capacity to generate, shape and disseminate information compels us to grapple with a critical question: Who or what now commands the mastery over our shared narratives, cultural discourse and collective understandings?
The Illusion of Objectivity
There is a widespread expectation that AI can serve as a neutral, emotion-free and purely data-driven observer. However, this very anticipation reveals a subtle human yearning for objectivity, a desire to believe there exists some immaculate vantage point immune to bias. We often hope AI might deliver what we ourselves struggle to maintain: a crystal-clear lens through which reality can be perceived. However, is this dream of mechanical neutrality little more than our own projection?
Paradoxically, the more we rely on AI to establish objective truths, the more we risk overlooking our own responsibilities. We might assume neutrality naturally emerges from the computational rigor of algorithms, but true neutrality demands unremitting human engagement: constant questioning, critical dialogue and ethical decision making. A purely data-driven approach left unscrutinized can mask the covert influence of biases embedded in the data itself.
Omissions and Power Dynamics
Imagine a student employing AI to research global historical narratives. The system confidently presents a coherent summary, replete with dates, places and key figures, yet on closer inspection it leaves out critical cultural perspectives. Entire communities, events or viewpoints may be missing or significantly underrepresented. The student, if sufficiently inquisitive, might notice these omissions and ask why they occurred.
This gap prompts reflection reminiscent of Michel Foucault’s philosophical inquiries into power and knowledge. Foucault asked whether truth can ever be the neutral product of reasoned discourse. His answer suggested truth is far from a simple end goal of objective inquiry; it is embedded in cultural conventions, institutional structures and, above all, in power relations. Like Humpty Dumpty’s insistence on mastery, Foucault warned that whoever controls the discourse often controls the framework through which truth is perceived. AI’s seemingly neutral outputs then are not neutral at all. They can reflect power imbalances especially those encoded in the training data just as readily as any other product of human culture.
The Skinnerian Lens: Conditioning and Behavior
The philosopher and psychologist B. F. Skinner adds another dimension to this conversation. In asserting that environmental conditioning shapes human behavior through reward, punishment and repeated habits, Skinner challenged the conventional notion of free will. If our behaviors, beliefs and modes of thinking stem from our environment, our biases are not random quirks or even fruits of logical thinking but outcomes of systematic and often invisible processes of conditioning.
When we consider AI from a Skinnerian vantage point, we see that AI’s biases are in large part reflections of our own. The algorithms do not become biased in a vacuum; rather, they learn from data that we curate. In other words, AI mirrors us back to ourselves. This interplay highlights the need for philosophical humility: Though we might aim to build unbiased systems, we must accept that our own biases and our historical patterns of thought shape those systems.
This recognition reframes bias, not merely as a defect to be stamped out but as an intrinsic feature of human cognition an inevitable lens through which we perceive the world and define meaning. A bias-free AI, one capable of perfectly accounting for every conceivable cultural viewpoint or historical context, is not only practically unattainable but conceptually problematic. Even if we tried to incorporate endless nuance, the sheer volume of information would overwhelm us, limiting its usefulness.
Acknowledging Bias Responsibly
In light of the impossibility of absolute neutrality, our ethical responsibility shifts. Our goal can no longer be the total eradication of bias, since that is neither realistic nor necessarily desirable. Instead, we must strive to acknowledge bias openly, manage it responsibly and intervene when it perpetuates harm or entrenches power imbalances. Transparency, the process of explicitly stating the perspectives and limitations behind both AI systems and human decision making becomes a moral imperative.
However, transparency without genuine accountability risks trivializing bias. If we merely confess our biases and move on without any corrective measures, we may normalize dominant narratives under the veneer that all perspectives have bias. Such complacency can perpetuate existing structural inequities. Thus, while openly acknowledging bias is a step in the right direction, it must be followed by deliberate efforts to highlight and examine marginalized perspectives.
When done right, admitting bias and taking corrective action builds trust. In academic, corporate and other communities, transparency can open the door to collaborative solutions. It invites multiple stakeholders to challenge assumptions and to cocreate systems that are more inclusive. This process, despite being messy and iterative, underscores an important lesson about humanity itself: We progress through acknowledgment of our differences and the willingness to address them head-on.
AI and the Possibility of Inclusive Futures
One of the most significant ethical opportunities AI poses lies in its capacity to analyze vast, diverse datasets. Properly guided, AI might unearth patterns that help us appreciate different historical narratives, cultural expressions and social phenomena. In theory, AI could provide a mosaic of global voices, giving unprecedented prominence to those who have been systematically excluded from mainstream discourse. However, this hopeful vision only becomes reality if we have the collective will to make it so. If we train AI systems using narrow, homogenous sets of data, or if we deploy them with profit motives that ignore minority perspectives, we only recreate existing inequities on a broader scale.
Here again, the tension Michel Foucault’s ideas raises becomes palpable. The question is not just whether AI can be harnessed for good but whether the institutions deploying AI have a vested interest in promoting inclusivity. Are we willing to reshape educational, political and commercial structures to ensure AI projects address systemic inequities? Or will we allow these technologies to reinforce the established hierarchy of voices?
Ethical Agency: A Deliberate Choice
If we adopt Skinner’s perspective that our beliefs and behaviors arise from environmental conditioning, then genuine ethical action demands conscious, deliberate efforts to push back against inertia. Power dynamics and institutional structures will not spontaneously yield more just outcomes. In practice, ethical progress often arises from resistance by activists, educators, policymakers and ordinary citizens who recognize bias and work actively to counter it.
This conception of ethics is neither automatic nor guaranteed. It emerges when we exercise moral courage: the resolve to scrutinize ourselves, to confront institutional partialities and to use AI in a way that fosters genuinely inclusive knowledge creation. In the realm of AI, moral courage can manifest as questioning algorithmic outputs, championing transparent data governance or advocating for diverse teams of developers and researchers.
Consistency of Ethical Vigilance
We should also note an inconsistency that dogs human history. While some technologies, especially AI, undergo rigorous ethical assessment, other areas of life (such as economic policymaking or military decisions) do not always benefit from equally intensive moral scrutiny. We see budgets, strategies and geopolitical moves that shape the fate of millions, yet ethical oversight is often cursory or fragmented. This uneven application of moral principles begs the question of how committed we truly are to addressing bias, power and accountability wherever they appear.
However, if the conversation AI’s biases sparks motivates a broader demand for ethical consistency, we could witness a shift with far-reaching implications. By constantly emphasizing the role of power, data, and cultural narratives in shaping AI’s outputs, we become more attuned to the ways these same forces affect myriad other spheres of life. The lens we develop for AI can in turn illuminate biases in governance, social structures and everyday human interactions.
Reclaiming Our Role as Master
Humpty Dumpty’s assertion that the real question is “Which is to be master?” remains startlingly relevant. Our technologies have become indispensable, yet we must not abdicate ethical judgment to machines or assume that an algorithm can transcend bias entirely. Instead, we must be active participants, conscientious stewards and humble caretakers of the tools we build. In a world where perfect neutrality is illusory, the best we can do is embrace our responsibility with eyes wide open.
AI’s biases reveal more than just technological shortcomings; they highlight the intricacies of human cognition and society. They force us to confront the limits of our own perspectives and the environments that have conditioned us. Acknowledging these biases can paradoxically expand our moral agency. If we accept that we will never have the final, unbiased truth, we also accept a challenge to continually improve, to strive for more inclusivity and to remain open to multiple voices and experiences.
And finally, AI prompts us to revisit timeless philosophical debates about free will, power and the very nature of truth. By reflecting on these issues, we recognize our ethical obligation to become proactive custodians of AI, guiding its development and deployment in a manner that elevates rather than diminishes our collective humanity. In a reality where absolute neutrality can never be truly attained, our moral mandate is clear: We must unite self-awareness, humility and concerted action to ensure these powerful systems serve equitable and inclusive ends.
References
Carroll, L. (1872). Through the Looking-Glass, and What Alice Found There. Macmillan.
Foucault, M. (1977). Discipline and Punish: The Birth of the Prison. Pantheon Books.
Skinner, B. F. (1971). Beyond Freedom and Dignity. Alfred A. Knopf.