Published on
Why and How to Build a Learning Analytics Community of Practice
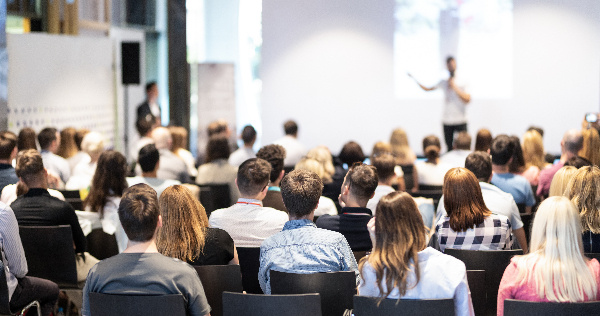
Recently, I joined colleagues from other institutions for a conference panel presentation about our experiences building communities of practice around the use of analytics. The use cases varied from academic to administrative outcomes involving students, faculty and staff, but a common thread was how to use and build community focused on leveraging data to better understand and improve decision making. My contribution was about learning analytics (LA), so I’ll focus here on why and how an LA community of practice (CoP), especially among faculty, can help students learn and succeed. To do so, I’d like to briefly describe our CoP origins but then let the definition of the CoP itself make the broader case.
Analytics at UMBC
Over the past 20 years, UMBC has developed a reputation as a thought leader in higher education on the use of analytics for institutional effectiveness. We’ve also worked closely with other institutions on the ethical and transparent use of data to democratize everyone’s access and understanding. And since 2021, our Division of Information Technology (DoIT) and Office of the Provost have collaborated to support a UMBC learning analytics CoP. The result has been more than 20 recorded webinar-style presentations, mostly by 22 LA fellows who propose and work on interventions to improve student success in exchange for a one-year, $2,000 professional development stipend that is renewable. Notably, one LA fellow, Karen Chen (IS), won UMBC’s 50th NSF Career Award based in part on her project: Learning Analytics by Students for Students.
To be honest, we totally stole the LA CoP idea from Indiana University, based on its wonderful Center for Learning Analytics and Student Success (CLASS) and LA Summit, at which I and others from UMBC have attended and presented. In turn, several from IU have presented at UMBC LA CoP meetings, especially when we were first getting started and hadn’t yet graduated enough faculty LA fellows to present their work. One of the key architects of IU’s CLASS and LA fellows program was George Rehrey (now retired), who coauthored a wonderful 2017 learning analytics and knowledge (LAK) conference paper for which I just happened to serve as a reviewer.
The problem George and others were trying to solve is that often the very people meant to benefit from analytics—students and faculty—are seldom engaged or involved in the design, implementation and evaluation of analytics interventions. That resonated with me, as I’ve seen the same. Sometimes analytics is a top-down administrative priority, with little or no engagement of the intended beneficiaries. So, I’d like to answer why a learning analytics CoP makes a difference by defining its implicit value proposition and methods.
Disclaimer and Goal
To be clear, purporting to analyze and improve human behavior, let alone learning, is both bold and inherently challenging to prove. Despite our best efforts and intentions, human learning is a wonderful but perplexing mystery. For this reason, the initial hype surrounding learning analytics (eerily similar to what we’ve seen with MOOCs, maybe even AI now) has faded, and some have even quibbled with the term “learning analytics” of all humans in favor of analytics of specific learners in specific contexts. “The teaching and learning research space is much more complex than we realize," says Vince Kellen, CIO at UC San Diego, in describing the first of his Seven Commandments of Learning Analytics—cultivating humility. “Large jumps in teaching productivity akin to 20th-century technology is not likely."
And yet, we still persist in trying to improve human learning because, in one way or another, we’ve all probably helped or benefited from the reality of actually doing so, elusive as it may be to define. For our purposes, however, I would argue that the primary goal of all educational institutions, teachers and courses is (or ought to be) helping all students honestly and accurately assess what they currently know, understand and can do—in any context—then figure out how to close any gaps in where they see themselves vs. where they’d like to be. Basically, we should want to scale self-assessment, so students become self-regulated, self-directed and thus lifelong learners after they leave our hallowed halls. As such, let’s now turn to our second term, “analytics,” to define how it can help.
Actionable Intelligence
There are many ways to define analytics, but actionable intelligence may be the best. For example, if you had to predict one outcome for current students based on what you think you know or understand about prior ones, could you do so? If so, could you act on it (i.e., intervene) to change the predicted outcome? If not, why? Do you need to know or understand more about current students to effectively intervene?
Basically, analytics implies going beyond mere analysis—a similar but very different word—to actually proposing, implementing and evaluating relevant interventions—a key concept in the term’s earliest definitions (Brown, 2011; Long & Siemens, 2011; Prinsloo & Slade, 2017). In short, analysis that does not lead to action, ideally with results that can be further analyzed and further acted upon, is not analytics. Put more simply, analytics without action is just analysis.
Why is action so important? Apart from the impact on intended recipients, interventions make explicit what those who implement them value implicitly about a desired outcome. For example, if students are skipping class, some may understandably focus on tracking attendance as an intervention to compel them to come and (here’s the implicit assumption) pay attention. But the root word of attendance—attend—does not guarantee attention, as some have found by allowing or banning student mobile devices.
In short, actionable intelligence makes outcomes more visible for all to see, critique and adapt to their own context. When it comes to learning analytics, it’s easier to infer and scale what works when we can see it—or think we can. And inevitably, when it comes to nudging student learning or, perhaps more importantly, identifying effective course design practices that help, it’s easier to do so together. Why? In my experience, faculty learn best from other faculty, which leads to our next term: “community of practice.”
How Democratizing Data Can Change Culture
As former UMBC president, Freeman A. Hrabowski, III, used to say, “If you want to change the culture, shine light on success, not failure.” By giving everyone a bird’s-eye view of campus data, analytics makes it easier to identify and reverse-engineer effective practices correlated to different (higher?) levels of student engagement. But identification and celebration of exemplars also invite private reflection and self-assessment among faculty about their own teaching practices, which can be the engine of pedagogical innovation.
For example, in 1999, Douglas Robertson proposed what is now considered a classic model for how faculty beliefs about teaching influence their evolving pedagogical practice; it includes the following stages:
- Egocentrism—focusing mainly on their role as teachers
- Aliocentrism—focusing mainly on the role of learners
- Systemocentrism—focusing on the shared role of teachers and learners in a community
If this evolution of belief and practice occurs among teachers, Robertson identified telltale signs of the transformation. First, as faculty move from one stage to the next, they bring the benefits and biases of the previous stage. Second, they typically change their beliefs and practices only when confronted with the limitations of a current stage, which is brought about by "teaching failures". Finally, their desire for certainty, stability and confidence either keeps faculty frozen in a status quo framework or drives their evolution into the next stage in an effort to avoid a paralyzing and stagnant neutral zone consisting of "a familiar teaching routine that they have deemed inappropriate and with nothing to replace it".
I believe any CoP informs all these stages but particularly the last one: If you’ve honestly and accurately concluded that what you’re doing isn’t working—again, a self-assessment skill and mindset we wish to instill in students—then you either double down and dig in with your status quo, or you start to look elsewhere for inspiration. A CoP among colleagues and peers can help identify, address and assess common pedagogical problems that all faculty face in designing and implementing courses to aid student learning and success.