Published on
How AI and Language Model Technology Can Accelerate Educational Excellence
Brooks Doherty | Associate Chief Academic Officer, Rasmussen College
Dee Kanejiya | Founder and CEO, Cognii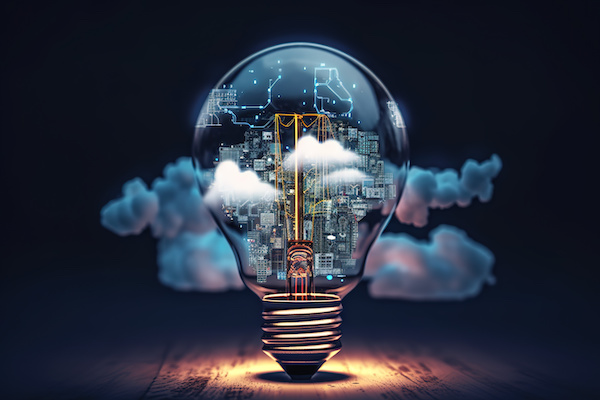
A collaboration perspective from an academic excellence leader and an AI innovator on the rapidly changing AI and Language Model field and its impact on the education industry.
As the Institute for the Future has written, “The human story is one of using technology to extend our senses and ourselves.”
The relationship between learners and faculty remains at the center of higher learning—both now and deeply into the future. However, the possibilities of artificial intelligence to enhance this relationship by providing student support and assessment capabilities are expansive, fascinating and require deep individual and organizational thought.
There are already examples of artificial intelligence services drawing upon universities’ student support data to answer transactional questions about, for example, financial aid or library support as easily as asking Alexa or Siri for the time. Additionally, AI can propel universities into more advanced assessment capabilities—beyond portfolios and multiple choice—as AI is able to accurately evaluate complex authentic assessments that reflect the demonstration of skills in real-world or closely simulated settings. In other words, AI, working alongside our faculty’s teaching expertise, can consistently and accurately assess the valuable knowledge our adult learners are bringing to our classrooms.
The line that separates the classroom from the so-called real world is already blurring. Increasingly, learners carry their experiential knowledge into the classroom and conversely bring what faculty teach them immediately into real-world practice. The faster and more accurately we can assess this learning and support this new learner, the stronger the relationship between learners and faculty and the greater our 21st Century Skills will be adopted.
There is also the question of the ethical use of generative AI—by students, faculty and universities. It appears that any AI worth its code will be able to outrun any plagiarism checkers with a few simple adjustments to how it presents the vibrancy of the language it generates. As such, it is incumbent upon universities not to run away from or ban the use of generative AI but rather to deeply consider how this technology will alter its assessment, teaching, learning and business models. The first step is to understand how these tools work and the impact on the human learning experience.
AI and Language Model Technology
Artificial Intelligence has been an active area of research since the 1950s with the goal of mimicking human intelligence exhibited across different cognitive faculties such as language and vision. A language model (LM) is a mathematical model for primarily assigning a probability distribution over a sequence of words or symbols. Since the 1980s, language modelling research has witnessed multiple paradigm shifts from deterministic to statistical to neural architectures as a higher order structure for predictive tasks in speech recognition, machine translation and question answering. The currently popular large language models (LLM) use artificial neural networks that allow extensive interactions between words through multiple layers of vector transformations and can be trained in a generative manner to predict a text sequence or an image. These LLMs have become feasible due to the availability of large amounts of training data.
How to Develop an AI and Language Model Strategy
LLMs have found a great utility in developmingvnatural language based conversational interfaces or chatbots that generate a longer response, as exemplified by OpenAI’s ChatGPT and Google Bard. They have often demonstrated superior and highly creative performances in generating a linguistic or visual response to a user’s prompt or question, which has led to their rapid popularity among consumers as well as enterprises now considering developing an AI strategy for organizational excellence and future readiness. LLMs can be a productivity-enhancing tool for human-mediated content generation or analysis tasks such as generating a draft of a blog post, a lesson plan or an artistic image, or summarizing a meeting. LLMs have also received a fair bit of criticism as their responses are not always accurate and may sometimes contain factual errors or hallucinations. Reliability, controllability, bias, optimization for educational use and inference costs are some of the factors to consider when developing an AI and language model strategy.
An AI strategy’s successful outcomes also depend on how well an AI service provider is mission-aligned to your organization’s core priorities. A good AI partner can help you navigate the rapidly changing AI landscape, provide in-depth technical expertise on how various AI models work, develop custom and proprietary AI solutions for your unique requirements, and help build trust between your AI solution and your end users. When implemented correctly, an AI and language model strategy can significantly improve an organization’s quality, efficiency, productivity, scalability, return on investment, and market competitiveness.
The challenge for colleges and universities will be the capacity to evaluate and update their learning assessment strategies in the context of LLMs. Artificial intelligence will not be the end of reading and writing, just as it did not kill the game of chess, but it will drastically change how we read and write. (It is not the first technology to do so, e.g., the pencil, the ink pen, paper, the Word document, spell check, text dictation and the list goes on.) Universities may need to diversify their student learning assessments from over-reliance on essays, discussion posts and other assignments, which would be easy for LLMs to complete without a great deal of human thinking or input.
How Rasmussen Implemented AI Technology
In 2021, Rasmussen University’s educational innovation team looked into designing and developing innovative Prior Learning Assessments for general education using Cognii’s conversational AI. These assessments present a student with a unique scenario and ask them open response questions.
After familiarizing themselves with the scenario, a student constructs a natural language answer by writing a short or long paragraph to demonstrate their critical thinking and problem-solving skills. The language model used immediately evaluates the textual answer for accuracy and generates a proficiency score and qualitative feedback that prompts the student with additional information. The feedback is designed to be in the zone of proximal development to bring out the best of the student without giving them the answer. The student responds to the AI feedback by updating their answer to further demonstrate their proficiency.
This type of conversational assessment leads to more active engagement between a student and AI in a pedagogy known as ‘assessment as learning’ which is closest to how real-world assessment takes place between humans. This also reduces the stress normally associated with taking a test, and at the same time generates deeper pedagogical insights about students’ internalized knowledge, comprehension, linguistic expression, learning progression, resiliency, and fine-grained conceptual mastery.
Recommendations for Higher Education
The Rasmussen University project with Cognii is an example of how, through partnership, higher education may explore different assessment models to prepare for a future of learning in which generative AI and LLMs are as ubiquitous as spell check. To prepare for this future, we recommend:
1. Organizational education
Some leaders in your organization have likely obtained a deep learning of LLMs and the potential impacts of AI on students and learning. It is just as likely that others have only been exposed to this technology through popular media. Educate the organization with dedicated time, resources and discussion before leaping into policies and practice.
2. Thoughtful policies
Some in your organization may want immediate policies surrounding generative AI—to have it banned or to rush out and purchase the latest ChatGPT plagiarism software. Use the dedicated time and discussion to understand the impacts of these policies and investments, and how rapidly the technology can change, before making decisions that may be difficult to unwind.
3. Put students first
It may be tempting to spend all your time discussing the robots, but never lose sight of the potential benefits to students. Don’t just focus on plagiarism and academic integrity. How can LLMs help students for whom English is a second language? How might they revolutionize tutoring and library research? Energize your organization with the potential benefits to students while being prepared for challenges.
4. Be aware of the rapidly shifting market
The generative AI market and the tools it offers seem to be shifting literally every day. Leverage AI leaders’ expertise to understand these changes and evaluate their applicability to your college or university. As technological advancement accelerates, be cautious as well as participatory by implementing innovative pilot projects to maintain a competitive edge.
Much of the innovation literature over the past generation has taught us that significantly disruptive technologies change markets, and those working in the market need to be prepared and work from a place of realistic hope rather than fear. Generative AI and language models may be the latest—and most significant—disruptive technology of this and the next generation. We must use this moment to extend ourselves, not retreat into the past.
Author Perspective: Administrator