Published on
Institutional Flexibility and Student Support Rooted in Data and Analytics
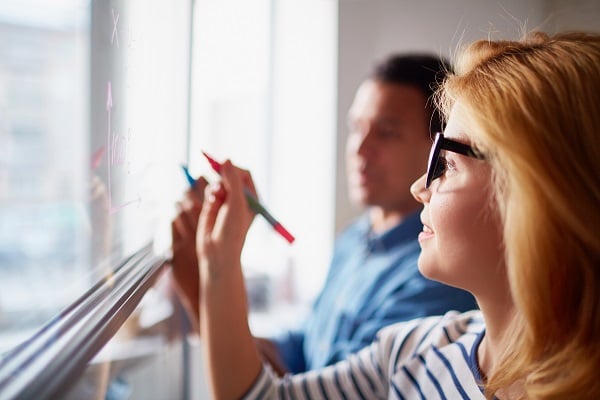
As a CIO at a private Catholic university in the upper Midwest, it is very clear to me that the future success of the University of St. Thomas depends in part on our ability to incorporate new skill sets in Big Data and analytics. While these two terms have been used to describe a general approach to finding hidden patterns and trends in large data sets, I am using them more broadly here to include machine learning, deep learning and artificial intelligence (AI).
At St. Thomas, we have changed a great deal in a relatively short time (at least, by higher education standards). In our not-so-distant past, we were the College of St. Thomas, a small liberal arts Catholic school that served only male students. Today, we are the largest private institution in Minnesota, a co-ed, national university ranked by US News and World Report.
However, what has gotten us to this point will not be sustainable moving forward. Minnesota’s demographics are changing rapidly, and our traditional pool of Catholic, white, often suburban Twin Cities students is not only declining, but also subject to increasing competition (not only from other Minnesota schools but also from those in our surrounding states). Replacing this decline is a rapidly growing first-generation and immigrant student population, bringing rich cultural and ethnic diversity along with new challenges for student success and advising staff.
What does this mean for us? We have an outstanding opportunity to live out our mission of serving the common good by educating this emerging student population. However, we can’t just apply our standard practices to these new students and hope that they will be successful in our environment. What is needed is a way to unearth patterns and trends that will:
- Help recruit the students that are a match for our institution, and
- Help these students succeed over the short and long term.
The current best-practice approach to this problem is still valuable: Have outstanding data scientists sort through data on the student information system, the learning management system, and the customer relationship management system (along with many other associated systems), and find actionable insights that can help change institutional approaches for advancing student outcomes. What does it mean when a student suddenly registers for a large overload in credits? What if their meal fund becomes precariously low? While these things may lead to early alerts at some institutions, the specific items may have far less meaning at other schools.
This approach is also great for finding patterns that get in the way of student success. Are there certain sequences that make graduating on time easier or harder? Is there a pattern that explains the success of certain at-risk students where others struggle? How do online offerings impact the success rate for certain kinds of working students?
Over the next few years, machine learning will become increasingly important to incorporate into an institutional data and analytics strategy. We already see Amazon’s Alexa, Google’s Assistant, Apple’s Siri, and Microsoft’s Cortana being rapidly adopted around the world, and higher education will not be an exception. Some schools are already experimenting with using deep learning and AI to discover and act on their institutional data. For example, Deakin University in Australia uses IBM Watson to uncover micro-patterns in the data and immediately turn them into actions to help promote student outcomes. According to their CIO, William Confalonieri, their “Genie” app will launch soon, answering smartphone texts from students 24 hours a day. It will know almost everything about the students’ academic lives: their grades, their majors, the classes they are taking, and even their campus activities. Deakin’s “Genie” will be a trusted, always-there advisor for these students, using data and analytics analyzed through deep learning approaches to provide an AI-based virtual assistant.
Finally, I think collaboration will become essential, especially for smaller institutions. I have been in conversations with my peer Twin Cities CIOs to work out ways to collaborate on similar big data/analytics/machine learning initiatives at St. Thomas. The masked data that we could pool together might provide deeper insights into student success factors than we would find working alone, and the ability to pool sourcing and valuable skills in this space could help us to create a new ecosystem of systems, APIs, data practices, and technology talent. While we may occasionally compete for the same students, I believe that we have far more to gain from working together than by fighting small battles.
Author Perspective: Administrator