Published on
How to Increase Staff Efficiency with AI Tools: 5 Key Takeaways from Online Adult Education Leaders
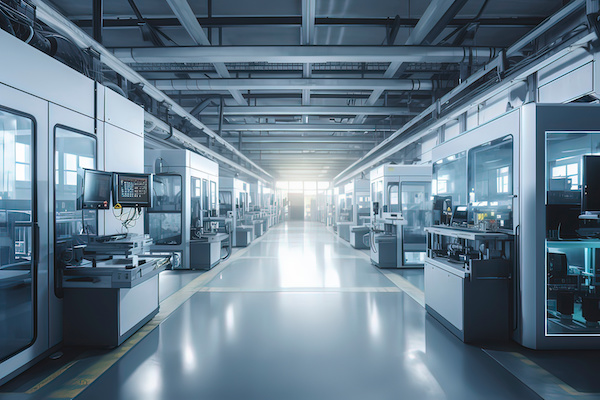
With the rise of generative AI tools, it’s almost impossible to avoid hearing about all the possibilities they present and what they’ll be able to accomplish in the future. But in the world of education, the demands to figure out these tools—both for ourselves and our students—are more urgent.
We’re already seeing surveys showing that around 40% of students already use these tools. As educators, whether in traditional higher education, Continuing Education or another adult learning environment, we need to raise the average level of comfort and familiarity with AI—not just to keep up with our students but also to improve productivity and staff efficiency, make scaling education more effective and ultimately give educators more time and resources to spend building relationships with students.
For this article, the folks at Ribbon Education talked to three leaders in online adult education—Pete Barth, Chief Product Officer at Flatiron School; James Genone, VP Academic Solutions and Innovation at Minerva Project; and Tade Oyerinde, Chancellor at Campus, as part of their “AI in Adult Education” webinar series. Here are their key takeaways, best practices and tips for implementing AI tools with their teams and increasing staff efficiency with AI.
Get Your Whole Team Involved
Tade: One thing we’ve seen be really effective in increasing staff efficiency and adoption of AI tools is just getting everyone involved from the very beginning, really from the ideation process. We did an AI Day, where we gathered the whole organization together for a half-day to test out a lot of tools people had seen or in which they were interested.
For people who hadn’t tried these tools yet, it was a great, low-pressure way to get introduced to them. But we were also able to have a whiteboard session around the best use cases, how we could incorporate them into workflows and so on. That was great because, instead of these ideas being a top-down imposition, team members who were really excited about them—who’ve spent hours and hours testing and playing around with them—got to share these ideas and learnings with the broader team. It was so much more effective that way.
James: We’ve done something similar at Minerva. We did a workshop where we worked with very open-ended questions and ideas for how we could use AI in our work and had everyone experiment together in real time to start finding answers to those questions. Then we gave our team the homework of going out, trying things on their own, then sharing in Slack what they found was working and what they were learning.
Experiment to Find the Best Use Cases
Pete: The best use cases for AI aren’t always obvious right away. So, I think giving your team space and time to experiment and see what’s going to work and what’s actually going to improve efficiency is critical.
For example, we often need to take our current body of curricula and create the first draft for a new engagement. We’ve found that using AI drastically reduces the amount of time it takes to get that first draft. Of course, you still need domain experts to edit, craft and shape it appropriately, but it does make a measurable difference. So, I think it’s crucial to allow your team to experiment with different tasks and processes—especially frequently repeated ones—and see how AI might help or might not.
Tade: Right. It’s not going to be perfect. We’ve experimented a lot with our engineering team, and AI’s not yet good enough to help with programming core business logic or something like that. You still need a team that can think through the architecture of what you’re writing. But there are many parts of programming that most engineers just don’t enjoy. They don’t want to write unit tests and manage debugging and so on, but AI can automate a lot of that.
AI is really good at automated testing, automated debugging and that sort of tidying up. By finding those ideal use cases, we’ve had high adoption because it’s automating processes that engineers aren’t really enjoying anyway. And now, all our engineers are shipping about an extra 10% to 20% of high-quality code just by using AI automation for some of these processes.
When You Find Something That Works, Document It (and Share It!)
James: Another best practice I’ve found is creating a document where I keep certain patterns of prompts that work really well for repetitive tasks. That way, I can easily come back and search for prompts that have worked well in the past, but I can also try them in different AI tools or models and see what works well where.
Pete: Many folks on my team have done something similar, creating scripts to help generate prompts that have all the right contextual information for curriculum work. They’re running their context and work into a script that actually helps them build the prompt that feeds into the AI tool. It’s important to remember that finding the best use cases isn’t just about determining what to use AI for but also determining which tools to use, who should use them and how we can use them most effectively.
James: We’ve also been making an effort to create some documentation around best practices we can use internally or that our partners can use. We want to create internal best practices and help our team understand how to use these tools and what makes them most helpful. But we also want to help university leaders think about AI strategy and create a tactical guide for improving course design using AI or thinking about how to get students to use AI thoughtfully instead of mindlessly. And we’re working on more technical documentation, too. Right now, it’s more just best practices—finding what’s working, yes, but also documenting that and sharing it with our team and our partners.
Spark Curiosity
Pete: The most important part right now is curiosity. Increased efficiency will come when leaders and staff have the time and space to play around with these tools, to get curious about them and to experiment and see what works. I see our role as hiring and encouraging that curiosity to find people who want to play with these tools and understand how we can use them to become more productive.
And students will also need to be curious about these tools and experiment with them too, so one of our jobs as educators is to help develop that curiosity.
James: Right. We just need to recognize that people are in very different places right now when it comes to their familiarity with these tools. There are people who, for example, have barely used ChatGPT at all, and others are power users and trying new AI tools every week. So, the question is, How do we not limit the creativity of people as they explore new uses while also sparking curiosity from folks who are less familiar without expecting too much from either group?
Tade: Agreed. Engineers, for example, might all be at least vaguely interested in this kind of technology. But outside of that group, you’re not always going to have this same culture of tinkering or curiosity about AI. So, we have to figure out what the right on-ramp is for those different groups, and that’s going to be a key factor in increasing adoption across the board and ensuring we’re improving efficiency across our entire teams with these tools.
Pete: One thing we did in one of our town halls was conduct fun AI exercises that, rather thannot asking how we could use this in our work or classroom, asked things like how we could we use it to tell a child a bedtime story or how to use it to plan a vacation. And then we just played around with several text-based and image-generation tools. Giving everyone the opportunity to dabble helped spark some of that curiosity and just to take the edge off as well.
Don’t Limit Yourself To Just ChatGPT
While ChatGPT is the best known and most talked-about AI tool right now, there are dozens of other user-friendly, specific and helpful tools out there that make use of AI. Some of our favorites include:
- Github CoPilot (for coding help and generation)
- Midjourney (for image generation)
- Replit Ghostwriter (for code generation)
- Supernormal (for meeting note and to-do generation from automated meeting transcripts)
- Anthropic (an AI assistant for managing tasks at scale)
Conclusion
Humans are always going to be great at certain things—for example, getting students excited about the content and motivating them, ultimately building relationships with students to hold them accountable. With the rise of AI tools and technology, the key is being thoughtful about using them to free up instructor and staff time, so they can spend more time on things like building relationships with students, motivating them getting them excited to learn and shaping their perspectives.
This technology not only has the potential to supercharge teachers and all learner support staff but also allows us to scale resources to students who need them the most to create more equity in the education field.
Ultimately, AI won’t just affect learners in the classroom and along their educational journeys. It also has a wide-ranging impact on basically everything—from future employment to remote education possibilities, learner competencies, classroom design and more. Staying ahead of the curve and figuring out ways to integrate it into workflows, student interactions, classrooms and coursework now will only help your team become more productive and provide more value to learners who are going to eventually be expected to be fluent in these tools.
This is the first part of a series of events on Harnessing AI in Adult Education. If you enjoyed reading this recap, consider RSVPing to the upcoming live events.