Published on
Beyond Security: Overcoming Additional Risks Associated with Institutional Data Analytics
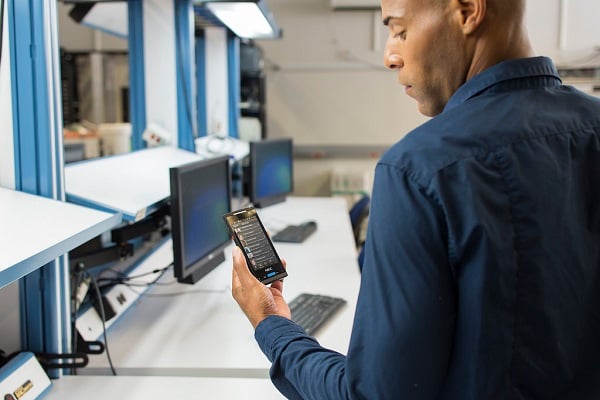
The advantages of leveraging Big Data in the higher education context are becoming increasingly clear to college and university leaders. From helping to drive student persistence and success to informing strategic and tactical management decisions, analytics are changing every aspect of the operation of postsecondary institutions. However, as more data is collected and parsed, the risks associated with this practice must also be considered and addressed. In this interview, James Wiley reflects on some of the most significant risks that come with leveraging data analytics and shares his thoughts on how institutions leaders can work to overcome these obstacles.
The EvoLLLution (Evo): Why are more and more colleges and universities turning to Big Data and analytics to support institutional management?
James Wiley (JW): There has been a lot of confusion around what constitutes Big Data—many people think Big Data just means having a lot of data but actually there are other aspects to it. Big Data is defined by the three V’s. There’s volume, which is the quantity of data. There’s variety, which describe the different types of data sources. Finally, there’s velocity—the speed the data moves—which is critical. Other people have added a fourth V called veracity, which speaks to the truth and accuracy of the data.
Institutions now are under a lot of pressure to prove their value, to ensure that they enroll the right students, to ensure they retain their students, and to prove that their students are learning. That is driving a lot of the inquiry around data; institutional leaders want to know what’s going on outside the institution—in terms of what’s happening in the workforce—and they want a better understanding of what’s going on within their walls.
These challenges around whether institutions are delivering value, retaining students, and supporting learning outcomes are increasing awareness of the criticality of bringing Big Data on campus.
Evo: As analytics and Big Data become increasingly central to the effective management of colleges and universities, what new risks are institutions becoming vulnerable to?
JW: The first big risk to leveraging Big Data is privacy and security. To really understand this issue, we need to look past the commonly discussed security of the data when it’s “at rest”—when it’s stored in the given system. Though that is a very valuable consideration, and leaders should ensure their systems are secure and certified, there are three additional risks that are often overlooked.
The first major risk occurs when the data moves. There are a lot of integration platforms out there to help connect the various systems—SIS, LMS, customer lifecycle management, etc—that institutions have running on campus. In fact, one thing that’s helping analytics out is the ease of integration between systems that’s now available. However, when the data is moving, leaders have to be absolutely sure that it’s encrypted and that access is authorized at either end.
The second major risk is the unintentional release of Personally Identifiable Information (PII), which has a number of diverse consequences. There are aspects of the algorithms and models being used by institutions today that can unintentionally release information that you can use to infer the identification of a student or contribute to the unfair treatment of a given student if your model has some bias in it. This leads to other questions around how good any given model truly is—whether it is predictive or descriptive—when it comes to protecting the identity of students and also in showing there’s no bias.
The third major risk is around the quality of the data itself. One big problem institutions have is, though they’re collecting data across numerous systems, if the data is not efficient, of good enough quality, timely or complete, the output may be flawed. As such, leaders have to learn the risks of making decisions based on flawed inputs. As such, it’s critical that leaders think about the quality of their data on the input side. This speaks more broadly to the quality of management processes in running every system in such a way that inputs are useful.
Evo: How useful is data when it’s not integrated and shared campus wide?
JW: Siloing between divisions and not integrating data across a campus risks the validity of the data across the entire enterprise. I have seen institutions that have 10 different divisions all operating independently, where there are different last names for the same student listed in different systems. It’s only by surfacing those data that you realize something’s not quite right and then you have to make policy decisions based on that.
This is an important point: Driving institutional data integration is not necessarily a technological decision. It’s a policy decision. There are institutions that still use multiple systems to manage different divisions independently of one another and that’s fine as long as the main data is being kept and managed in one system. However, it’s very important to begin to validate that data across different systems to find some conflicts and other differences that will be very important to consider when doing analytics.
Evo: How would not addressing these risks impact institutions over the long term?
JW: There are a few potential ramifications from not taking steps to address these risks with leveraging Big Data. One is litigation and lack of compliance. An institution could secure a system for a particular regulation like HIPPA, but then if they move the data to, or integrate the data with, another system that doesn’t have the same compliance levels the institution might be out of compliance and then, as a result, possibly face litigation. So compliance and litigation is one area leaders have to consider as they’re moving data from various systems into an analytics engine.
The other issue is basically developing the wrong picture of a given student, or releasing a student’s information, which may result in a compliance issue but might also lead to the development of misinformed policy. In some cases, if I have a model that shows a particular sub-group that keeps underperforming and I’m taking action on that, creating interventions to support this sub-group’s success, and it turns out that my model is flawed and/or my data is presenting the wrong information, I’ve unfairly painted that sub-group as in need of intervention. That could cause some unique issues on its own.
Evo: What happens when an institution doesn’t focus attention and resources on the quality of the data they’re collecting and the implications of the algorithms that they write?
JW: When a leader is presented a picture that is not correct, they begin to create strategy and design interventions aimed at solving a problem that might not actually exist. If the data quality is wrong and that output is incorrect, leaders wind up devoting resources in the wrong area, and it might not even address the problem at all.
Evo: What key steps must institutions that collect, store and analyze large amounts of data take in order to protect that information from outside (and internal) attack?
JW: The major thing is for IT to first separate out what belongs to tech and what belongs to policy. From a tech perspective, institutions can make sure that data is encrypted, can ensure that data at rest is secured and can put in place an SOC (service organizational control) to manage other risks.
On the other side, there are policy decisions about quality around privacy that must be addressed. For example, what’s the minimum number of students required to make a data analysis valid? That’s one policy question that defines the development and understanding of sub-groups; it’s critical make sure that the minimum size isn’t too tightly drawn that I release student data unintentionally. Answers to policy questions come about through iterations in the data and testing with different models to see data could be unintentionally released.
The second thing, once IT and policy responsibilities are defined, is identifying risk points. This requires leaders to look across their entire analytics ecosystem—all the sources being brought in, all the data sets being used—and identify specific risks that come up when folks try to answer specific questions. For example, a member of staff might need to access secured data and move it into an unsecured system to be able to answer a specific analytics question. Those issues need to be addressed.
The third step is to prepare everyone for this conversation about data governance and data quality. Leaders have to get everyone in the room to identify data stewards and to make sure everyone in the institution understands their responsibilities with data.
Evo: What are a few roadblocks IT leaders can expect to face when trying to take these steps, and how can they be overcome?
JW: The first thing leaders need to do is get their arms around how data is approached across the institution and understand where and how the data is stored. Oftentimes at institutions. the acquisition of data systems or applications is decentralized. Different divisions, be they advancement or enrollment management or anyone else will go purchase something that suits their specific needs—the process is completely decentralized. All IT is concerned with is whether each team’s system is secure and whether it will cause any problems. But now that we’re in the analytics era, leaders need to truly get their arms around all the systems operating on their campus, find out what’s contained within them and ascertain each system’s quality of data.
This brings us to the second part of the issue, which is around ownership and organization. Who owns the data? Who’s responsible for managing it? Who are the data stewards? One organizational problem might be that no one is responsible for managing data quality. A team might have a lot of people who input data and take out data, but there’s no point person accountable for making sure that this data is of sufficient quality or is secure.
Evo: Is there anything you’d like to add about what it takes to really ensure that an institution is capable of using the data that it needs to drive effective institutional management?
JW: When a lot of institutions start with Big Data, they’re not sure what it is they want to solve. Leaders don’t know what they don’t know, so they just bring in truckloads of data and then plan to analyze it to determine what more they need to know. This approach is ineffective.
Institutions should begin with some specific, critical, strategic questions they are trying to answer. Is it about enrollment patterns? Is it about intake or yield? Is it around student learning? Defining the questions you’re trying to answer, and identifying those questions up front, helps to organize and streamline the data analysis process. That’s part of the policy portion of this, and it would help IT to prioritize their efforts and start the process of assessing what they have and what they need to be able to answer the questions at hand.
Ultimately defining a strategic question as a starting point will help focus everyone’s attention on the critical questions, get buy-in and help the IT team prioritize its efforts on the backend to make sure that those data and those systems are up to speed, secure, integrated well and that the data is private.
This interview has been edited for length and clarity.