Published on
What Will Be Important in the Learn and Work Ecosystem in 2030? How Do We Prepare?
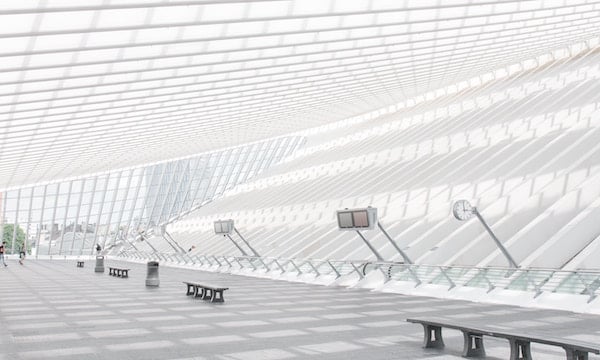
To help tackle these questions, Lumina Foundation recently sponsored the second of two convenings on AI Future Skills with the Institute for the Future (IFTF), an organization that has been forecasting ten-year futures for 50 years. IFTF has a well-tested foresight process, which we followed. We convened a diverse group of thinkers and practitioners and asked them to think about what will go away in 10 years, then think about what will be most important. We ran four scenarios, painting four different pictures of what 2030 might look like based on research about the drivers of change. With each scenario, the group considered what actions would be needed should that scenario prevail.
This October, IFTF will issue a report on the convenings, which included experts in artificial intelligence, education, economics and workforce. The report will summarize the experts’ views on what skills will likely be needed to navigate the learn-and-work ecosystem over the next 10 to 15 years, and their suggested steps for better serving the nation’s future needs.
Here are a few of their predictions about life in 2030:
- Computing speed will increase, and massive computing space will be available, no longer dominated by systems maintained in the cloud as “edge computing,” which will enable analytics and knowledge generation to occur at the source of the data. There will be significant growth in algorithmic thinking (allowing solutions to be automated by using sets of instructions or rules that, if followed, will enable thinking with many levels of abstraction). With all of this computing power, we will be overburdened with data “noise” as algorithms migrate to the precise sources of data.
- Most people will live in approximately 20 “megacities” or regions. Our population will be diverse but polarized, marked by an aging population that is predominantly white, and a large young population that is largely non-white. Resource allocation will be strained as these differing populations essentially compete for services. People will live longer, and the working-age population will represent a broader spread; learners will begin learn-and-work programs at younger ages to optimize opportunities for growth, and many will work well past their mid-60s, though workplaces are expected to change significantly (as will definitions of “work”).
- Many advances in technology and science will affect our lives, from synthetic biology, to low-cost—or even free—DNA sequences, to robotic assistants at home and in workplaces. Sensors will personalize the world of “things”—for example, sensors in cars, coffee mugs, and home appliances.
- The complexity of the issues we face in a data-dominated world will compel us to form more open and innovative partnerships.
- Human performance will be augmented by AI. As machines become increasingly recognized as citizens with “rights,” policies about access and fairness will undergo drastic changes. Artificial reality and virtual reality tools will also accelerate learning in education/training systems.
- There will be serious challenges from cyber- and bio-terrorism, less human control over our environments, and potentially uninhabitable environments in some locations. These challenges will require new know-how, flexibility and coping skills. Humans will suffer serious psychological impacts as they face environments over which they have less control.
This context-setting sets the stage for exploring four future scenarios through the lens of a worker/learner (scenarios will be described in the October report). For each, we considered the same questions: What are the skill sets that will serve this future? What are the new vulnerabilities and new risks? What, if anything, is the safety net? What are the new opportunities? What action steps should be taken today in light of this scenario?
While each scenario generated actions specific to that scenario, some actions appeared in all four scenarios. This suggests it may not be as important to be able to precisely predict the future. Rather, it is more important to look at the main signals, trends and drivers of change, and determine the actions that need to be taken no matter the scenario.
These seven suggested actions—common to all scenarios—especially resonated with Lumina:
- Focus on learning: All learners will need a range of competencies and skills, most critically: learning how to learn; having a foundation in math, science, IT and cross-disciplines; and developing the behaviors of grit, empathy and effective communication.
- Prepare all “systems”: Schools will continue to be important places to teach competencies and skills. Parents will be important teachers for children. Workplaces will also be important places for learning, and many learners will need instruction on how to work effectively as part of human/machine teams.
- Integrate education and work: Education systems will need to be integrated with work in an education/work ecosystem. To enable movement within the ecosystem, credentials will be useful, but only if they are transparent and portable. The competencies and skills that stand behind credentials will need to be identifiable, using a common language to enable (a) credential providers to educate/train for an integrated education/work system; (b) employers to hire people and upgrade their skills; and (c) governments (federal/state/local) to incentivize and regulate programs and policies that support the education/work system.
- Assess learning: Assessing competencies and skills acquired in multiple settings and modes (including artificial reality and virtual reality tools), will be essential. AI will enable powerful new assessment tools to collect and analyze data about what humans know and can do.
- Build fair, moral AI: There will be a high priority on ensuring that AI has built-in checks and balances that reflect moral values and honor different cultural perspectives.
- Prepare for human/machine futures: Machines will join humans in homes, schools and workplaces. Machines will likely be viewed as citizens with rights. Humans must prepare for side-by-side “relationships” with machines, especially in situations in which machines will be managing aspects of education, work and life formerly managed by humans. Major questions will also arise about the ownership of AI structures—what ownership looks like, and who profits from ubiquitous AI structures.
- Build networks for readiness/innovation: Open and innovative partnerships will be needed for whatever future scenarios emerge. In a data-rich world, we won’t solve problems alone; networks, partnerships and communities will be key.
One action seemed to summarize best what we need to do to prepare for the future: focus on learning. We will all need to be learners throughout our lives. How learning will occur and what learning will be valuable are questions that still loom. We don’t have to predict the future precisely; multiple scenarios are possible. Still, we must prepare for the changes that are coming. For Lumina, that means providing opportunities for all learners to learn.
Author Perspective: Analyst