Published on
The Death of Content as King: How a Data Democracy Has Revolutionized Marketing
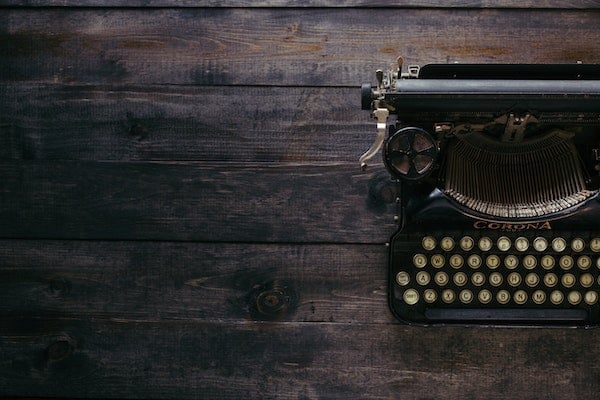
This article is excerpted from The Death of Content As King: How a Data Democracy Has Revolutionized Marketing (2020).
CHAPTER EIGHT
Building a Data Democracy
Continuing education and professional programs have been a hallmark of Washington University in St. Louis since 1908. Founded officially in 1931 as the professional and continuing education division of the College of Arts & Sciences within Washington University, University College has been serving the St. Louis region ever since. University College offers more than forty programs of study, with programs at every level. These include associate, certificates, undergraduate degrees, post-baccalaureate certificates, advanced certificates, and graduate degrees helping students advance their careers. All students can explore new fields, while growing personally and professionally. However, by 2016, when I arrived as the director of marketing and communications, University College faced serious issues. Enrollments had been declining by about five percent for several years.
Most of the people living in St. Louis were unaware of the availability of programs at University College. From my viewpoint, I had worked professionally in the St. Louis market the majority of my adult life, while being unaware of University College. I worked specifically in higher education marketing for several years. While aware of Washington University, I was still unaware of the University College division until I applied for the job. I was a part-time student in the evening for both my bachelor’s and master’s degrees. But still, I was unaware that University College had these types of programs, at least as I studied at another university less than forty miles away. I was a veteran student, so University College offered the Yellow Ribbon Program that would have made tuition free; yet, again, nothing. It was clear to me that University College struggled with a lack of brand awareness within the St. Louis market and an undefined relationship with Washington University. With over a dozen brick and mortar competitors in the region, thousands more online in the marketplace were crowded and noisy. The yearly marketing budget for University College wasn’t big enough to outspend the competition, and so I knew we had to find a smarter approach.
The problems facing University College weren’t new. Raising brand awareness and finding more students was a common issue facing the modern university, including continuing education divisions. At first, I approached the problem by looking at the conversion attributions of each part of the student journey at the research and discovery stage. Students would request information from the website or attend an open-house night. However, the traditional open-house event relied too heavily on finding one evening that fit into the schedule of a busy adult. Here digital marketing could increase registrations for the event, but it couldn’t guarantee attendance would follow. Meanwhile, the website was organically creating the same amount of inquiries each month as an open house. The lack of brand awareness at passive and active exposure stages made the cost of trying to get new students to directly apply for a program and skip inquiring caused diminishing returns. With more than forty programs at University College across multiple levels, there wasn’t enough budget to do program-specific search marketing for every program. The solution wasn’t going to be found by just addressing the marketing of the student journey.
When the marketing and admission teams started to look at the data side of the student journey, we found the opportunities that were being missed. We had access to enrollment information that was at the end of the journey. So, we could see the demographics of University College’s students in individual courses. We hadn’t been excluding Washington University employees using tuition benefits for their own education. This meant we were including an audience that wasn’t degree-seeking, taking fewer courses per semester, and had irregular enrollment patterns. A better understanding of University College’s audience was needed to find new degree-seeking students. We started by building data-driven personalities of our audience, which combined data points from several sources. Website analytics monitored key locations on our website for degree programs, applications, and conversion points. Audience intelligence tools than separated web traffic from these potential students into a variety of demographic, behavioral and psychographic data segments, which was indexed against population size in the St. Louis region and proclivity to convert. From here, we could overlay these segments against first-party data in our application management system and CRM. The resulting personas were the most accurate view of our students to date. This enabled us to find marketing solutions that were better targeted. Messages resonated because it was the right audience, and students were more interested in degree programs we offered. Within just three short years, the enrollment trend was reversed, improving three to four percent across consecutive semesters.[1]
Starting a Data Revolution
No democracy is formed overnight, so neither is one built on data. Starting a data revolution requires more than just having the data points or the understanding of how to use them. It requires alignment that brings together data silos across organizations and stakeholders. It drives purpose towards a meaningful impact to the consumer who is sharing with the organization. Establishing a foundation can be both flexible to any data set for a product or service. Still, it is maintainable without needing unlimited resources.
Just because the marketing department wants the data doesn’t mean other departments and their stakeholders are willing or required to share it. While it might make sense in a hierarchy-based organization to just convince the leadership at the top to order other departments to share access to their data silos, this approach rarely finds happy stakeholders in enthusiastic compliance. Stakeholders need to understand the purpose and goal behind building the data democracy together to get buy-in. It is important to remember that stakeholders are not going to simply rely on logical arguments, as fellow human beings would. Instead, it must be an emotional appeal that solidifies the alliance. Stakeholders can’t be positioned as losing control of their data silo. How the data flows must give reverence to the sourcing systems and departments.
The purpose behind building a data democracy shouldn’t be just to have one or to just collect data with no reason. Every data point should work towards an end goal as the collection for which will require the cooperation and benefit for the consumer. Even if the consumer is not an active contributor to the democracy, once they are aware passively of the collection, their reaction will be based on the perception of who benefits. Should the organization be taking advantage of the arrangement, the consumer might abandon or reject the product or service in the future. Conversely, if the consumer sees the advantage in their favor, they might be less resistant to providing additional data and consider the benefit an extension of the product or service under consideration.
It might sound over the top to consider a constitutional convention as a method in which to establish a foundation for a data democracy. Yet, a project this important to an organization shouldn’t be conceived on the back of a napkin after work at the bar. The inclusion of stakeholders will require an agreement that can be reviewed and amended to reach consensus. End goals need quantifiable metrics to measure success that respects the consumer providing data. In this way, they must benefit from an equal member of democracy. The framework should also look to be limited enough in scope to be manageable by all departments involved. This requires a data model that constantly provides feedback and can be responsibly maintained beyond the tenure of the founding department leadership.
VOTE Data Framework Model
Every industry has unique metrics that matter only to their marketing goals, while at the same time, sharing other metrics across different product or service sectors. Rather than attempt to assume which metrics will matter to all marketers and organizations when building a data democracy, a framework model is a better approach. With a model that focuses on what data should mean to an organization, marketers can avoid data overload and useless reports that display only vanity metrics. Therefore a relevant model for data democracy should only contain data that is valuable, organizable, targetable, and explainable (VOTE).
Valuable data is any meaningful and relevant for an organization’s goals. Data in this category is the most salient when it can be connected between the consumer and the product or service. The intent here is that not all data is valuable to an organization’s, and should be used to make the amount of data manageable for all stakeholders. The discovery of valuable data is possible when its presence is justified by both quantitative and qualitative factors. Does having more of this data improve the model? Does having this data provide insight into the consumer journey or audience? Only data that is positive in both factors should be considered valuable.
Organizable data has the most criteria in the model as it is only data that can be collectible, storable, sortable, and repeatable. Just because marketing wants the data doesn’t mean the consumer can or will share it. To be collectible, the consumer must be willing and able to share it. When the consumer shares that data, marketers must have a location to store it for as long as needed. From there, they must be able to sort it in relation to all other data in the model. The collection of the data should be repeatable so that the model remains representative of consumer insights, while always collecting more data points to improve.
Targetable data should be data points within the model used for directly aiming or indirectly knowing that for which consumers aim. The intent is to remove any doubts for marketers about what data is needed or required by this model. Without data that is targetable, nothing in the rest of the model is useful to an organization. Targetable data should answer the who, what, where, when, and why of consumers. At the same time, it provides insight into how to reach them. Marketers having targetable data know that they are including the right data in the model, because again, both the quality of the data and quantity of the data should improve the targeting on the consumer journey.
Explainable data is the criteria that any data used in the model is understood by stakeholders within and outside of marketing. Every organizational hierarchy marketing is accountable to others higher up in leadership, and so, they need to understand why the data is useful to marketing. If the data isn’t created or stored by marketing, the other departments need to understand why it is important for marketers to have those data points. This means more than just providing a data definition. The quintessential reason for marketing is to “show their work” to prove that all the data in the model is valuable, organizable and targetable. By taking the time and resources to make sure the data is explainable, the framework for the VOTE model will sustain the data democracy for its duration in support of the organization’s products or services.
Responsible Democracy
An often retold story after the Constitutional Convention in the United States was when founding father Benjamin Franklin was asked what type of government had been created. His answer was, “A republic, if you can keep it.”[2] A data democracy faces similar challenges. Once it is planned and built, it will need to be maintained. Neglect from mentalities like, “set it and forget it.” This will only lead to ruin that can devalue the quality of data and can lessen the quantity of data points available. Therefore, establishing guidelines for data maintenance that includes hygiene, append, purchasing, and unification will help in both keeping this important democracy and enabling it to thrive.
The practice of data hygiene is the maintenance of data points by cleansing unusable and inaccurate data. This helps improve the accuracy and targetability of the data model, increasing its efficiency over time. Marketers familiar with direct mailing tactics have used address hygiene tools from the postal service and certified mail service providers for decades. Email marketing platforms and CRMs have automated controls for similar maintenance and prevention. However, good data hygiene practices shouldn’t be limited to just outbound efforts but rather an essential activity within every part of the data model.
It is very likely that not all the data points that are needed will come from a single source. The use of data appending is necessary to join different sources into a single data view. Considerations should be given to what sources of data can overwrite different data points and in what direction will each append occur. Data sources closest to the consumer should have priority while likely also providing improved insight. Caution should be taken when appending, since that might risk the chance of mixing valuable data with irrelevant data points, or that an automated append process might overwrite useful data fields. By following best practices, data appending can enhance data models with greater depth that can widen consumer understanding. Thus, it improves how the overall model is used.
The limit of most data models is what data can be collected from the consumer by the organization, however with data purchasing, third parties can provide an expanded data set from their sources. The important difference between these sources is that while third-party data is aggregated data on a general group of people, first-party data is information the marketer collects directly from the customers like their interactions with the organization. If third-party data lets the marketer reach a broad persona, then first-party data lets them pinpoint a hyper-specific persona.[3] Data models should not rely exclusively on data purchases or data from sources that come from third parties, but rather they are a way to enhance first-party data and data points provided directly from the consumer. Typically the approach to appending and purchasing data will likely flow in a single direction, while the best approach for any data model should be the unification across all data sources and views. This should allow for the two-way flow of data across all sources in the data model. The optimization that can flow from unification can also improve data hygiene by purging all sources of duplicates and useless data points. This is not to argue that data democracies should start by attempting to gather all the data. Remember the VOTE model, but take the best data points from the best sources together into a unified view of the consumer for personalization.
With the above guidelines in place, an organization has started the process towards establishing data governance. While the term might have a negative connotation for some marketers and communicators not wanting to lose control, the choice is a preemptive move against certain worst-case scenarios. Leandro DalleMule, chief data officer at AIG, and Thomas H. Davenport, President’s Distinguished Professor in Management and Information Technology at Babson College, a research fellow at the MIT Initiative on the Digital Economy, and a senior adviser at Deloitte Analytics, writing for the Harvard Business Review points out that, “The importance of investing in data governance and control—even if the payoff is abstract— is more easily understood and accepted if a company has suffered from a major regulatory challenge, a data breach, or some other serious defense related issue.”[4] There are many dangers to any democracy, but addressing these vulnerabilities early with stakeholders builds trust in helping preserve a bright future for a data democracy.
Greetings, Programs!
At this point, marketers and communicators have both what to consider as a framework when starting a data democracy and how to maintain that data model in the best interest of the organization and its stakeholders. The cornerstone that is missing is where the data should go, which data tools should be considered, and how that will impact the consumer. This is becoming more imperative to organizations. In most cases, data is becoming a differentiator because many companies don’t have the data they need. Although organizations have measured themselves in systematic ways using generally accepted accounting principles for decades, this measurement has long been focused on physical and financial assets — things and money.[5] And yet, what makes the most powerful platforms Google, Facebook, and Amazon? They all continue to be how they’ve accumulated, organized, and used their data.
Of course, not every organization has the capacity, resources, and time to emulate the major platforms in building self-sufficient data democracies. However, some platforms are designed to help businesses collect and manage data. Popular customer relationship management (CRM) services like Salesforce and Microsoft Dynamics along with enterprise resource planning (ERP) platforms like Oracle and SAP are widely considered industry leaders in helping organizations manage both products and services with data. Many more CRMs, ERPs, and marketing tools exist. The above brands shouldn’t be considered an endorsement of any service over another. But rather, considerations should be given to outside platforms that are designed for these purposes over the internal development of in-house software. Vijay Gurbaxani, Founding Director of the Center for Digital Transformation (CDT) and Taco Bell Endowed Professor of Business and Computer Science at the Paul Merage School of Business, University of California in Irvine writes in the Harvard Business Review about the platform economy of how online marketplaces are changing the face of business. He wrote that, “An important consequence of the platform economy is that, other things being equal, bigger players often do better than smaller ones. When a larger business invests in a software platform, the gains accrue from a greater number of customers, employees, or production units, which means that it can justify an investment when a smaller competitor cannot. This means smaller businesses need to build their software platforms for scale right from the start. Since scaling quickly is tough, a smaller business may need to create partnerships or networks to help them grow. Either way, scale becomes a critical part of how companies employ software thinking. Leverage scale if you have it; build for scale if you don’t.”[6] This means that outside platforms offer better capacity. Scale for organizations from the start struggle in attempting to build in-house software unless that is the product or service the organization is trying to create.
Of course, not all software is created equal. Only true platforms that harness developer ecosystems to create third-party applications that enhance the platform or customization to fit different product and service sectors have the true market advantage in helping organizations build data democracies. This network effect of developers multiplies the impact of a platform that very few organizations can match with in-house developer teams. This is best illustrated with an example scenario, Company A and Company B. Both can both afford to hire five software developers, which according to Glassdoor, makes an average salary of $80,394 per year in 2019.[7] Company A looks to build their data democracy in-house from scratch, while Company B looks to take advantage of an outside platform that uses an ecosystem. Company A and Company B need their software to have five distinct functions. So, Company A hires five developers and assigns a single function to each of them. But now, they are out of developer resources. Meanwhile, Company B uses an ecosystem platform. Instead of hiring five developers, Company B hires only two while saving the other three salary lines. The ecosystem platform includes a community of consultants and developers, in addition to the modest amount of 100 developers working for the platform. This only costs Company B the equivalent of one developer’s salary because the platform has other organizational accounts. Company B can also select a consultant agency that specializes in building applications on the platform for their product or service sector. This agency alone has fifteen developers but only costs Company B two developer salaries because the agency can work for other companies in that sector. Company B now has 117 developers working to improve the same five distinct functions with the same cost in developer salaries as Company A that went in-house. Company A will always be limited by the abilities of their five developers, meaning that if they add functions, it either requires an additional developer or less time spent on the existing functions. Meanwhile, Company B can leverage 117 developers to either improve existing functions or expand into new functions. The developers working for the agency and the ecosystem platform also have more incentive to improve and expand functions. It will attract more companies to their platform and services, allowing each to hire more developers. Therefore, organizations that embrace a community driven approach of internal and external resources are better positioned to achieve cutting-edge data democracy than companies that silo their efforts into a single tribe.
Do Hackers Dream of Electric Sheep?
Cui bono, as the Latin phrase goes, “to whom is it a benefit” is the consideration that every data democracy must answer with a single word response: consumers. As in almost every way, the consumer interacts with a product or service both passively and actively. These activities belong to them. While data isn’t currently considered to be the property of an individual, at this moment in time, it is a transaction none the less. Therefore the consumer must benefit from the exchange of data that occurs between them and an organization for the product or service they have sought. The most important way to know if the consumer is benefiting or not is by looking at the product-market fit. The concept of product market fit began in technology startup culture. Growth hackers pushed the importance as a way to achieve what mattered most to startups that were ready to scale growth. Silicon Valley venture capital, Marc Andreessen, defined product-market fit to mean being in a good market with a product that can satisfy that market.[8] But that leaves the question of how to measure it from the consumer’s point of view. GrowthHackers’s founder, growth hacking author, and initial head of growth at numerous startups, Sean Ellis, considers product-market fit to be less abstract and measurable by asking a simple question. “I ask existing users of a product how they would feel if they could no longer use the product. In my experience, achieving product market fit requires at least forty percent of users saying they would be “very disappointed” without your product. Admittedly, this threshold is a bit arbitrary, but I defined it after comparing results across nearly 100 startups. Those that struggle for traction are always under forty percent, while most that gain strong traction exceed forty percent.”[9] From this metric and threshold, organizations can have an understanding of their product-market fit. Perhaps the first valuable data point for a growth hacking marketer and communicator to measure.
But what are growth hackers and why are they important to building a data democracy? According to Andrew Chen, the former leader of Uber’s Rider Growth product teams and now a venture capitalist, “Growth hackers are a hybrid of marketer and coder, one who looks at the traditional question of ‘How do I get customers for my product?’ and answers with A/B tests. They respond with landing pages, viral factor, email deliverability, and Open Graph. On top of this, they layer the discipline of direct marketing, with its emphasis on quantitative measurement, scenario modeling via spreadsheets, and a lot of database queries. If a startup is pre-product-market fit, growth hackers can make sure virality is embedded at the core of a product. After product-market fit, they can help run up the score on what’s already working.”[10] In essence, they are marketers that have embraced the possibilities of data utilizing skillsets reserved for software developers and engineers in order to enhance their marketing efforts. Perhaps if the data democracy is the engine in an organization’s car, then growth hackers are the all-in-one engineer that can build it. They are the salesperson that can sell the car off the lot, and the mechanic that can keep it running for years to come.
Growth hacking is more than just a job title or ability to use code in marketing efforts. It is a mindset. A focus on growth for the product or service that is informed by testing different marketing strategies and tactics, each test showing data points about what worked and what didn’t. This testing validates the attribution models that were the framework of data, marketing, and results that were key points of chapter two. Marketers and communicators learning with growth hacking techniques will understand which platforms like search from chapter three, social from chapter four, and/or e-commerce in chapter five. These can provide valuable, organizable, targetable, and explainable data needed in the VOTE model from this chapter. By embracing the “always be testing” philosophy,[11] growth hacking marketers can leverage every stage of The Modern Consumer Decision Making Journey in chapter six from initial consideration to retention and then perhaps subscription. Because skillsets in marketing don’t have to be limited to only writing generic content, the ability to include data in order to use techniques from chapter seven allows for personalization of content at scale. The wherewithal to see the whole board, understand how each piece of data works together, and then use those pieces to execute a strategy growth for the products or services that anticipates the consumers’ needs is exactly the kind of talent organizations need to build data democracies.
If You Build It, They Will Come
The concepts behind growth hacking and its utilization of data-driven models can be applied outside of startups to any category. This was true when I used these principles to growth hack UMSL’s CRM to increase the student enrollment funnel in 2014. In the years that followed, I improved the university’s website as a gateway for converting students and using social media marketing to drive sold-out open house events. The challenge was finding more ways to attract students with a marketing budget that hadn’t changed in the five years. The university wasn’t going to give us more money for another billboard, radio spot, or digital campaign. We had to find a no cost solution that would drive more students. But, we also had to understand more of why students chose UMSL. While UMSL asked students in the application process this question, as the car buying experience from chapter two demonstrated, the most common answer of “friend or family” wasn’t an attribution that we could model.
At this point, I remembered an article about a survey conducted by the University of California, Los Angeles (UCLA) which looked at over 190,000 first-year students across 238 four-year colleges and universities in the United States called the CIRP Freshmen Survey.[12] That year, UCLA listed the twenty-three reasons for choosing a college that was “very important” in influencing their final college selection. I reviewed the list from the top, looking for some insight into the student’s consumer journey of picking a college. The highest four reasons were: the college has a very good academic reputation (63.8 percent), the college’s graduates get good jobs (55.9 percent), I was offered financial assistance (45.6 percent), and the cost of attending the college (43.3 percent). None of these was anything the marketing and communication department at a university could impact directly. In fact, it would take any universities years to improve, if they even could. The fifth highest reason caught my eye, a visit to the campus (41.8 percent). We had already maxed out the open house events happening twice a year, but the campus tours were happening multiple times per day, every weekday.
We then started to build our data models looking for the most valuable campus tour related data from several key enrollment sources. We organized the information from the bottom of the funnel and worked up. The application had a campus tour data point that every student was answering. We could sort this data against the CRM of prospective students who were being imported or updated with a campus tour data source as having taken a tour. At the top of the funnel, we had website analytics on the campus tour website. Again, we compared this data to other sources for enrollment to see how often it was present in data records for students. Campus tour ranked sixth on a list of twenty-nine possible CRM touchpoints for UMSL enrolled students over two years, with half of the sources above it being automated touchpoints that every applied or interested student record would already have. It was undeniably a valuable data point. We also had a targetable data point to focus our efforts. We were looking for students who were interested in taking a campus tour to see the university for him or herself. Because we had taken the steps to determine if the campus tour data was valuable, we had organized that data point against all other sources. And because we could target this student from their initial visit to the campus tour website, we had all the criteria for explainable data sets for leadership to sign off on the project.
Still, the data was telling me this wasn’t going to be easy. The campus tour website had an eighty-six percent form abandonment rate. Those that completed the form had twenty-five total fields to complete. It was like the Energizer Bunny of forms. It kept going and going, scrolling across three separate full screenshots to capture it all. The easy solution would have been to just make the form shorter. In fact, that move alone could have increased conversion rates, but that wasn’t going to solve the problem. The campus tour website needed a better user onboarding experience. It had to allow for personalization, and a way to grow more campus tours from each student visitor.
My team at UMSL looked at user onboarding across a variety of sites, including the social network Twitter, the question and answer site Quora, and a now-defunct group dating website called Grouper. We realized that the best user onboarding experiences didn’t have one long form but were split across several steps. On the old form, the student’s name and contact info were first while the date of their tour was at the bottom. On the new form, we made this the first step. If the form was abandoned after the first step, we still knew who they were and when they were coming. We could contact them with emails to remind them and also send them back to the form to complete it. The next step helped them personalize their campus tour experience by speaking with an academic advisor, taking tour residence halls, or learn more about extracurricular activities like Greek life. There were over fifty different ways they could create an experience just for their interests. Again, if the student stopped at the second step, we knew who they were, when they were coming, and what they would like to see on campus. The next step in the form was the academic program and mailing address information that allowed for a more tailored communication plan before and after the visit. At this point, the student was completely done with scheduling their tour. Still, the form wasn’t done because a hidden bonus step appeared, asking if they wanted to invite up to two friends with them on the tour. One student signing up for a campus tour visit was good but getting three students at the same time was great. At this point, the student could enter basic contact info for their friends and the CRM would begin a communication plan to invite them on a tour with their friend. Now we could map the importance of the “friend or family” data point if we wanted, but more importantly, we had a more valuable personalized campus tour experience.
Within the first three months of the new campus tour website, we saw a thirty-seven percent increase in campus tours. By the end of the year, UMSL had the largest campus tour attendance in school history, without spending a dollar more in marketing. No single data source we used could have helped us reach this outcome. It required a data model that used the most valuable, organizable, targetable, and explainable data points that didn’t wait for students to tell us why they chose UMSL. It showed us their vote in the data.
This was an excerpt from Jon Hinderliter’s The Death of Content As King: How a Data Democracy Has Revolutionized Marketing, published in 2020.
Copyright © 2020 by Jon Hinderliter. Published by Tactical 16, LLC. Permission given for use of excerpt by publisher
References
[1] Gauen, Claire. “Serving Adult Consumers of Knowledge.” The Ampersand, April 25, 2019. https://artsci.wustl.edu/ampersand/serving-adult-consumers-knowledge.
[2] Beeman, Richard. “Perspectives on the Constitution: A Republic, If You Can Keep It – National Constitution Center.” National Constitution Center. Accessed December 6, 2019. https://constitutioncenter.org/learn/educational-resources/historical-documents/perspectives-on-the-constitution-a-republic-if-you-can-keep-it.
[3] Girard, Alex. “First-Party Data: How You Can Optimize Your Ads Targeting By Relying On Yourself.” HubSpot Blog, October 11, 2018. https://blog.hubspot.com/marketing/first-party-data.
[4] DalleMule, Leandro, and Thomas H. Davenport. “What’s Your Data Strategy?” Harvard Business Review. Accessed December 6, 2019. https://hbr.org/2017/05/whats-your-data-strategy.
[5] Beck, Megan, and Barry Libert. “The Machine Learning Race Is Really a Data Race.” MIT Sloan Management Review, December 14, 2018. https://sloanreview.mit.edu/article/the-machine-learning-race-is-really-a-data-race/.
[6] Gurbaxani, Vijay. “You Don’t Have to Be a Software Company to Think Like One.” Harvard Business Review, April 20, 2016. https://hbr.org/2016/04/you-dont-have-to-be-a-software-company-to-think-like-one.
[7] Glassdoor. “Salary: Developer,” October 1, 2019. https://www.glassdoor.com/Salaries/developer-salary-SRCH_KO0,9.htm.
[8] Andreessen, Marc. “The Pmarca Guide to Startups, Part 4: The Only Thing That Matters.” blog.pmarca.com, June 25, 2007. http://web.archive.org/web/20070701074943/http://blog.pmarca.com/2007/06/the-pmarca-gu-2.html.
[9] Ellis, Sean. “The Startup Pyramid.” Startup Marketing. Accessed December 6, 2019. https://www.startup-marketing.com/the-startup-pyramid/.
[10] Chen, Andrew. “Growth Hacker Is the New VP Marketing.” @andrewchen (blog), April 27, 2012. https://andrewchen.co/how-to-be-a-growth-hacker-an-airbnbcraigslist-case-study/.
[11] Taparia, Neal. “5 Things You Can Learn About ‘Growth Hacking’ From The Man Who Coined The Term.” Forbes, July 22, 2014. https://www.forbes.com/sites/nealtaparia/2014/07/22/5-things-i-learned-about-growth-hacking-from-the-man-who-coined-the-term/.
[12] Robert Morse. “Freshmen Students Say Rankings Aren’t Key Factor in College Choice.” US News, January 31, 2013. https://www.usnews.com/education/blogs/college-rankings-blog/2013/01/31/freshmen-students-say-rankings-arent-key-factor-in-college-choice.
Author Perspective: Administrator