Published on
Splitting Hairs: Exploring Learn-ing vs Learn-er Analytics (and Why We Should Care)
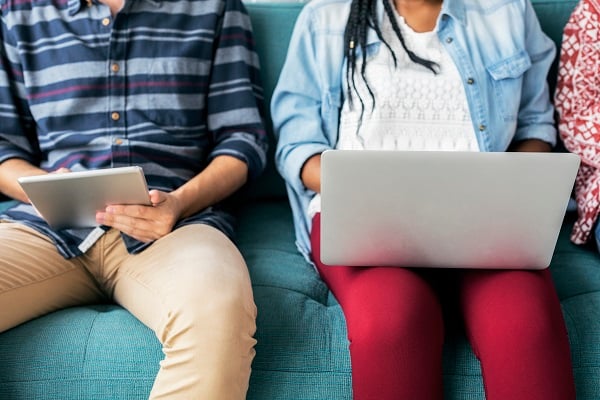
While it is certainly true that colleges and universities have been compiling numbers and generating reports for decades, the sheer volume of information now being collected as well as increases in computational capacity mean that much of today’s data use goes beyond mere reporting to become, instead, predictive and prescriptive. At the same time, the public outcry over college affordability coupled with accountability initiatives aimed at increasing effectiveness and efficiency demand a more analytical approach to data across all aspects of higher education. It is increasingly important that higher education understand what drives both their institutional performance as well as their students’ success.
In an effort to eliminate the “term sprawl” already occurring by 2011, Phil Long and George Siemens made the distinction between academic analytics and learning analytics. They defined academic analytics as the analysis of data to help monitor institutional success on key goals at the institutional, regional and international levels (such as effectiveness and efficiency across the institution, expanded capacity in STEM and college completion). They defined learning analytics as the analysis of data to help us understand the learning process (such as course- and departmental-level data to explore relationships among learner, content, institution and educator). The beneficiaries of academic analytics, Long and Siemens suggested, are administrators, funders, state legislatures, national governments and education authorities, whereas learning analytics mainly benefit learners themselves and faculty.
In this sense of the term, learning analytics focuses on how large-scale, fine-grained educational data might be used at the micro or course level to provide on-demand insights into how learning is progressing at any point in time. Learning analytics help students track their own activity and achievements at various levels of detail and help faculty understand what teaching methods and academic interventions are most likely to enhance learning of particular content and, even more specifically, with which learners.
While Long and Siemens’ distinction between the analytics we use at the institution level and the analytics we use at the student level was downright prescient at the time, there has been a lot of activity since then. In particular, the emergence of the relatively new category of “student success analytics,” which are often also lumped into discussions of learning analytics, has brought about additional “term sprawl” that Long and Siemens were attempting to avoid. In fact, by the end of 2016 EDUCAUSE noted that, since their original learning analytics report in 2012, the term had become “a complex topic that includes learner metrics (students’ knowledge absorption), matriculation-related success metrics, and the related systems and resources that contribute to learning and conventional measures of success” (p. 7).
The issue here is that this overly inclusive definition masks the fairly significant process and implementation differences between learn-ing analytics and what might be more accurately termed learn-er analytics, which fall somewhere between the Long and Siemens definitions of learning analytics and academic analytics.
As compared to learning analytics, analytics at the macro or “learner” level allow us to explore how the differences among learners affect their persistence and overall college success. Learner analytics is therefore concerned both with collecting information around differences among learners with regard to cognitive traits like aptitudes, cognitive styles, prior learning, and the like, as well as the learners’ non-cognitive characteristics such as differences in levels of academic motivation, attitudes toward content, attention and engagement styles, expectancy and incentive styles, personal experiences, affect, extra-curricular interests, socio-economic status and even family situations.
With these data, learn-er analytics attempts to predict things like which learners may have difficulty making the transition to college and identify the interventions best able support those at risk. Recent developments in the area of learn-er analytics have explored matching student characteristics to majors and career paths, increasing the likelihood they will remain engaged and persist through degree completion (see, for example, Degree Compass and Career Compass).
The reason this distinction may be important is in response to the growing conversation around how to change institutional culture to value and embrace the use of data to inform decision making and, specifically, in response to the question “how do we get faculty more engaged in using data to improve student success?”
To date, many of the analytics initiatives underway at our institutions—with the excellent support of vendors like Civitas, Hobsons/PAR, and EAB—are all focusing largely on learn-er analytics, which relies almost exclusively on the active engagement and coordination of administrators and administrative systems. And, while predictive analytics in this category have been leveraged to great effect in several notable cases, it has been possible to advance these learn-er analytics initiatives with minimal faculty involvement. And, frankly, it has been a lot easier for us to start there.
But achieving truly meaningful and sustainable change will require that we pivot and begin also exploring the promise of learn-ing analytics for our students’ success as well. This effort is going to involve an entirely different set of strategies because 1) learn-ing analytics more directly involves faculty and affects their roles on our campuses and 2) learn-ing analytics are a whole lot more complicated to interpret than analyzing “click stream” data and counting logins to the LMS. At the same time, learn-er analytics promises to continue advancing our institutions’ student success initiatives while also potentially becoming a key resource to initially inform curricular and instructional design.
So, while this may be splitting hairs, we have been using the term “learning analytics” to mean both learn-ing and learn-er analytics. But realizing the promise of these efforts is likely to require completely different strategies, stakeholders, expertise and cultural changes. It may be time to revisit our definitions.
– – – –
References
Arroway, P., Morgan, G., O’Keefe, M., & Yanosky, R. (2016). Learning Analytics in Higher Education. Research report. Louisville, CO: ECAR. Available online at: https://library.educause.edu/~/media/files/library/2016/2/ers1504la.pdf
Long, P., & Siemens, G. (2011). Penetrating the fog: Analytics in learning and education. Educause Review, 31-40.
1st International Conference on Learning Analytics and Knowledge, Banff, Alberta, February 27–March 1, 2011. Available online at https://tekri.athabascau.ca/analytics/.
Author Perspective: Administrator