Published on
Taking Advising Analytics Beyond the Numbers: The Road to Improved Academic Advising (Part 4)
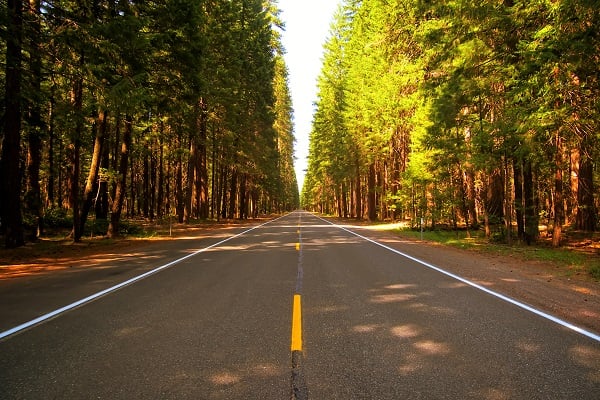
Discussion of analytics in higher education has reached a fever pitch. The anecdotal enthusiasm—and concern—is there, reflected in the number of articles written about analytics, conference sessions with it as a focus, and the increase in products and solutions aiming to support college and university analytics efforts. There is also quantifiable evidence of how much “analytics” is actually occurring on campuses. Over half of the 1,400 respondents surveyed in our report on advising practices and technology, “Driving Toward a Degree: Establishing a Baseline on Integrated Approaches to Planning and Advising,” said that both analytics and reporting solutions and early alerts, or analytics solutions that support identification of at-risk students, would have a positive impact on planning and advising effectiveness at their institution.
The adoption rates of these analytics solutions are also much higher than other tools, yet scaling this adoption across the institution remains a challenge. While 90 percent of institutions make at least some use of analytics and reporting at their institution, less than half consider use to be widespread. This difference is starker for early alerts solutions, with 84 percent reporting at least limited use of early alerts but just 28 percent reporting widespread use.
What are some of the challenges with implementing analytics such that tools are used across the institution instead of simply in pockets, and more importantly, how can institutions overcome these challenges? And what are some of the risks of new predictive analytics that can improve student success? The solutions to these challenges and risks are complicated, but below we offer a starting point.
Action Step 1: Get the Numbers Right
While institutions believe that analytics solutions can have an impact, many struggle with technical integration challenges. Our research identified institutions in one of four segments based on their progress with advising reform, and the Check Engine segment, representing institutions who favor advising solutions but report current technology use is ineffective, illustrates this dynamic best. Sixty percent of respondents in the Check Engine segment believe that technology, not people, has the greatest potential to improve academic planning and advising. Yet, 41 percent of respondents in this segment cite technical integration challenges as a barrier, significantly more than the 23 percent in the overall sample. Relatedly, Check Engines are the least likely of any segment to agree that technology used to support advising at their institution does a good job increasing effectiveness.
Integration is not the only way to improve analytics, but it certainly represents a good start. According to the Community College Research Center’s (CCRC) self-assessment “Evaluating Your College’s Readiness for Technology Adoption,” compatibility of new and existing IT is a key pillar of technological readiness. The self-assessment emphasizes IT’s need to share information across systems, lest end-users find themselves logging into multiple systems to find answers or repeat work inputting data. This seamlessness of information is critical to ensuring use of analytic solutions, because additional steps often make end-users less likely to adopt and use a tool.
In addition to integration, aligning the institution with a standardized data model is critical. The data model involves not only technical standards, but also alignment on common metrics and interventions. This last part requires change management and in Part Three of this series, we highlighted the need to involve a broader group of stakeholders, including advising leadership, in technology purchasing decisions. Institutions should maintain this involvement throughout solution implementation to avoid barriers to end-user uptake, driven by poor integration and inconsistent data models.
Action Step 2: Make analytics a “people initiative”
However, readiness for data and analytics in advising is not just about system integration and data models. Making the tool available, even if it operates smoothly with other campus systems, will not ensure that stakeholders will use the tool. Brian Hinote, Administrative Fellow in the Office of Student Success at Middle Tennessee State University (MTSU), echoed this when discussing the roll-out of Education Advisory Board’s Student Success Collaborative (SSC) at MTSU. He refers to advising reform initiatives, such as implementing the SSC predictive analytics solution, as people initiatives, not technology initiatives, and stresses project teams should be more concerned about stakeholder uptake of tools.
To ensure initiatives are people-focused, Brian emphasizes the importance of empathy and constant communication with stakeholders. For advisors and staff who were anxious or pessimistic about the SSC implementation, Brian set up meetings with them and allowed them to share concerns. In addition, Brian provided daily updates to faculty and staff after the roll-out of the tool, relaying the change requests his team had implemented. These actions showed stakeholders that his team was serious about their feedback and wanted the tool to make their job easier. Institutions who make data and analytics a people initiative, as Brian suggests, increase the likelihood of the adoption of tools, leading to an increased potential impact on student success.
Action Step 3: Use analytics to overcome stereotypes, not perpetuate them
Early-alert systems for advising allow institutions to use student data to target those students who may need assistance to stay on the path to a degree. Yet, institutions must be careful to ensure that the data does not perpetuate biases or violate student privacy. In her 2016 book, Weapons of Math Destruction, Cathy O’Neil discusses how big data, which should lead to greater fairness, in many cases results in the opposite outcome. Institutional leaders need to ensure that their adoption and application of early alert systems buck the concerning trend that O’Neil has flagged.
Institutions have access to data points that, while impactful as alert criteria, are sensitive for students and should likely not be used in isolation. For example, an advisor may be alerted that a student’s chosen major is too demanding based on their academic history, or that the student has underperformed in a core course for that major. Should that student be encouraged to switch paths, even if the major aligns with their career goals? Advisors cannot answer that question with the information from the alert alone; rather, they should use the flag as an opportunity to engage students in a discussion about potential implications and options.
Early alerts also raise issues of student privacy. New data forms not covered by the Family Educational Rights and Privacy Act (FERPA) are being used for Early Alerts, including student ID card swipes at certain locations including the dining hall or information sessions for student organizations, among others. Some experts have spoken out about excessive data collection, worrying that these tools invade student privacy despite what may be gleaned from the information.
Students should understand, at least at a basic level, what data will be used by predictive analytics systems and be able to opt out. And, at the very least, advisors and other faculty and staff who interact with student “risk scores” and other sensitive information need training and support to ensure data is handled properly and student recommendations are delivered effectively. For institutions employing predictive analytics systems, balancing student success with other factors such as bias and privacy will only get more complicated – so stakeholders should take steps to determine best practices now.
Tune in for next month’s post on student engagement, which will discuss insights and practical tips on getting students to adopt advising programs and solutions. For more insights and resources, including interactive data, on advising reform, be sure to visit drivetodegree.org.
Author Perspective: Analyst